Probability distributions on topologically nontrivial manifolds Announcing the arrival of Valued Associate #679: Cesar Manara Planned maintenance scheduled April 17/18, 2019 at 00:00UTC (8:00pm US/Eastern)HowTo: Method of Conjugate DistributionsInfinite fourth moment and maximum entropyNormal approximation with dependent variableswhat is the difference between statiscal averagre and average?Understanding a Probability TableWhat is a probability distribution?Set of all probability distributions supported on $Omega$ is a convex set.Probability Distributions, Continuity Corrections, Uniform Distributions, Etc.Is the following true: A distribution does not have a well defined mean iff its mean comes out as $pminfty?$Norm of Hilbert space Gaussian
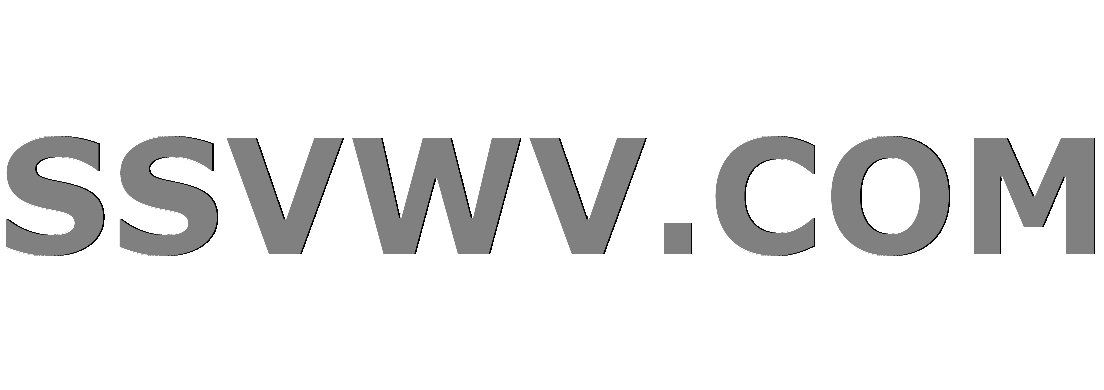
Multi tool use
How could we fake a moon landing now?
Why are there no cargo aircraft with "flying wing" design?
What do you call the main part of a joke?
Why didn't Eitri join the fight?
Where are Serre’s lectures at Collège de France to be found?
Is the Standard Deduction better than Itemized when both are the same amount?
Chinese Seal on silk painting - what does it mean?
How to find all the available tools in mac terminal?
What does the "x" in "x86" represent?
Do square wave exist?
また usage in a dictionary
How come Sam didn't become Lord of Horn Hill?
What does this Jacques Hadamard quote mean?
Can a new player join a group only when a new campaign starts?
Do I really need to have a message in a novel to appeal to readers?
Is CEO the profession with the most psychopaths?
When the Haste spell ends on a creature, do attackers have advantage against that creature?
Dating a Former Employee
Significance of Cersei's obsession with elephants?
Is it common practice to audition new musicians one-on-one before rehearsing with the entire band?
Do jazz musicians improvise on the parent scale in addition to the chord-scales?
What is the meaning of the simile “quick as silk”?
2001: A Space Odyssey's use of the song "Daisy Bell" (Bicycle Built for Two); life imitates art or vice-versa?
Why do we bend a book to keep it straight?
Probability distributions on topologically nontrivial manifolds
Announcing the arrival of Valued Associate #679: Cesar Manara
Planned maintenance scheduled April 17/18, 2019 at 00:00UTC (8:00pm US/Eastern)HowTo: Method of Conjugate DistributionsInfinite fourth moment and maximum entropyNormal approximation with dependent variableswhat is the difference between statiscal averagre and average?Understanding a Probability TableWhat is a probability distribution?Set of all probability distributions supported on $Omega$ is a convex set.Probability Distributions, Continuity Corrections, Uniform Distributions, Etc.Is the following true: A distribution does not have a well defined mean iff its mean comes out as $pminfty?$Norm of Hilbert space Gaussian
$begingroup$
I'm wondering if anyone could tell if there exists a widely accepted theory of probability distributions defined on topologically nontrivial manifolds? If so, as a physicist, I would appreciate providing some explanations using the, possibly, simplest example, a circle $S^2$.
Here are my thoughts. Generally, for a manifold $mathcal M$, I see no problem in defining some 'distribution' $f(x)$ with $xinmathcal M$, such that $int_mathcal M f(x)dmu(x)=1$. Obviously, this definition is metric-dependent. Still, oftentimes we have a canonical definition of the metric, e.g. borrowed from $mathbb R^n$ in the case if some canonical embedding is given, which is often the case.
However, we face serious difficulties when try to define 'averaged' quantities. (And in physics that's what we typically want to do).
Assume, given some 'distribution' $f(vecn)$, we want to calculate its mean value. One option would be to define it as follows:
$$
langle vec n rangle = dfracint limits_S^2 vecn f(vec n) dsleft
$$
The good thing about this definition is that it gives somewhat expected results, especially in the case of sharply-peaked distributions. However, we immediately face a huge number of problems. First of all, there exist a wide range of 'distributions' for which $langlevec nrangle$ is undefined (all the shells whose center of mass is at the origin). Second, excluding such 'bad' 'distributions' from the consideration does not really save us, for such an exclusion may be be 'quantity'-dependent (were we averaging not $vec n$ but smth else, we would have to exclude other distributions). Moreover, even if we exclude all the 'bad' ones (for a particular quantity of interest), we still cannot even define the sum for the remaining 'good' ones, for, again, the sum of 'good' distributions may be a 'bad' one.
OK, let's now consider a totally different approach suggested by the discrete probability theory. What is the mean value for the random variable which in a half cases gives $-1$, and in another half - $+1$? Well, clearly it's $0$, you would say. But wait, in terms of a 'discrete guy' who only deals with two objects in the universe, $-1$ and $+1$, this does not make any sense. There's no such object as $0$ in his universe. Nonetheless, this definition oftentimes makes sense. Why? Because we know that both $-1$ and $+1$ have a natural inclusion into $mathbb R^n$ where the mean value can be defined. Let us stop for a second and appreciate this fact - we allowed the 'mean' value of a distribution defined on the the set $mathcal S=-1,+1$ to take values on a different set $mathcalS' = [-1,1]$. (On the contrary, as of 03/2019, the canonical way of embedding heads and tails into $mathbb R^n$ is still not known, and, so, their mean value does not make much sense.)
Generalising this procedure to our example is straightforward:
$$
langle vec n rangle = int limits_S^2 vecn f(vec n) ds
$$
Which basically gives us a mean value of a distribution defined on $mathcal S'$ (again, by inclusion). An obvious downside - the averaged quantities have now no meaning for inhabitants of the $mathcal S$ manifold.
Is any of these approaches dominant? Or maybe smth else? Is there a theory for general, more complicated manifolds?
Any comments and /simple/ references are welcome.
probability probability-theory probability-distributions manifolds smooth-manifolds
$endgroup$
add a comment |
$begingroup$
I'm wondering if anyone could tell if there exists a widely accepted theory of probability distributions defined on topologically nontrivial manifolds? If so, as a physicist, I would appreciate providing some explanations using the, possibly, simplest example, a circle $S^2$.
Here are my thoughts. Generally, for a manifold $mathcal M$, I see no problem in defining some 'distribution' $f(x)$ with $xinmathcal M$, such that $int_mathcal M f(x)dmu(x)=1$. Obviously, this definition is metric-dependent. Still, oftentimes we have a canonical definition of the metric, e.g. borrowed from $mathbb R^n$ in the case if some canonical embedding is given, which is often the case.
However, we face serious difficulties when try to define 'averaged' quantities. (And in physics that's what we typically want to do).
Assume, given some 'distribution' $f(vecn)$, we want to calculate its mean value. One option would be to define it as follows:
$$
langle vec n rangle = dfracint limits_S^2 vecn f(vec n) dsleft
$$
The good thing about this definition is that it gives somewhat expected results, especially in the case of sharply-peaked distributions. However, we immediately face a huge number of problems. First of all, there exist a wide range of 'distributions' for which $langlevec nrangle$ is undefined (all the shells whose center of mass is at the origin). Second, excluding such 'bad' 'distributions' from the consideration does not really save us, for such an exclusion may be be 'quantity'-dependent (were we averaging not $vec n$ but smth else, we would have to exclude other distributions). Moreover, even if we exclude all the 'bad' ones (for a particular quantity of interest), we still cannot even define the sum for the remaining 'good' ones, for, again, the sum of 'good' distributions may be a 'bad' one.
OK, let's now consider a totally different approach suggested by the discrete probability theory. What is the mean value for the random variable which in a half cases gives $-1$, and in another half - $+1$? Well, clearly it's $0$, you would say. But wait, in terms of a 'discrete guy' who only deals with two objects in the universe, $-1$ and $+1$, this does not make any sense. There's no such object as $0$ in his universe. Nonetheless, this definition oftentimes makes sense. Why? Because we know that both $-1$ and $+1$ have a natural inclusion into $mathbb R^n$ where the mean value can be defined. Let us stop for a second and appreciate this fact - we allowed the 'mean' value of a distribution defined on the the set $mathcal S=-1,+1$ to take values on a different set $mathcalS' = [-1,1]$. (On the contrary, as of 03/2019, the canonical way of embedding heads and tails into $mathbb R^n$ is still not known, and, so, their mean value does not make much sense.)
Generalising this procedure to our example is straightforward:
$$
langle vec n rangle = int limits_S^2 vecn f(vec n) ds
$$
Which basically gives us a mean value of a distribution defined on $mathcal S'$ (again, by inclusion). An obvious downside - the averaged quantities have now no meaning for inhabitants of the $mathcal S$ manifold.
Is any of these approaches dominant? Or maybe smth else? Is there a theory for general, more complicated manifolds?
Any comments and /simple/ references are welcome.
probability probability-theory probability-distributions manifolds smooth-manifolds
$endgroup$
add a comment |
$begingroup$
I'm wondering if anyone could tell if there exists a widely accepted theory of probability distributions defined on topologically nontrivial manifolds? If so, as a physicist, I would appreciate providing some explanations using the, possibly, simplest example, a circle $S^2$.
Here are my thoughts. Generally, for a manifold $mathcal M$, I see no problem in defining some 'distribution' $f(x)$ with $xinmathcal M$, such that $int_mathcal M f(x)dmu(x)=1$. Obviously, this definition is metric-dependent. Still, oftentimes we have a canonical definition of the metric, e.g. borrowed from $mathbb R^n$ in the case if some canonical embedding is given, which is often the case.
However, we face serious difficulties when try to define 'averaged' quantities. (And in physics that's what we typically want to do).
Assume, given some 'distribution' $f(vecn)$, we want to calculate its mean value. One option would be to define it as follows:
$$
langle vec n rangle = dfracint limits_S^2 vecn f(vec n) dsleft
$$
The good thing about this definition is that it gives somewhat expected results, especially in the case of sharply-peaked distributions. However, we immediately face a huge number of problems. First of all, there exist a wide range of 'distributions' for which $langlevec nrangle$ is undefined (all the shells whose center of mass is at the origin). Second, excluding such 'bad' 'distributions' from the consideration does not really save us, for such an exclusion may be be 'quantity'-dependent (were we averaging not $vec n$ but smth else, we would have to exclude other distributions). Moreover, even if we exclude all the 'bad' ones (for a particular quantity of interest), we still cannot even define the sum for the remaining 'good' ones, for, again, the sum of 'good' distributions may be a 'bad' one.
OK, let's now consider a totally different approach suggested by the discrete probability theory. What is the mean value for the random variable which in a half cases gives $-1$, and in another half - $+1$? Well, clearly it's $0$, you would say. But wait, in terms of a 'discrete guy' who only deals with two objects in the universe, $-1$ and $+1$, this does not make any sense. There's no such object as $0$ in his universe. Nonetheless, this definition oftentimes makes sense. Why? Because we know that both $-1$ and $+1$ have a natural inclusion into $mathbb R^n$ where the mean value can be defined. Let us stop for a second and appreciate this fact - we allowed the 'mean' value of a distribution defined on the the set $mathcal S=-1,+1$ to take values on a different set $mathcalS' = [-1,1]$. (On the contrary, as of 03/2019, the canonical way of embedding heads and tails into $mathbb R^n$ is still not known, and, so, their mean value does not make much sense.)
Generalising this procedure to our example is straightforward:
$$
langle vec n rangle = int limits_S^2 vecn f(vec n) ds
$$
Which basically gives us a mean value of a distribution defined on $mathcal S'$ (again, by inclusion). An obvious downside - the averaged quantities have now no meaning for inhabitants of the $mathcal S$ manifold.
Is any of these approaches dominant? Or maybe smth else? Is there a theory for general, more complicated manifolds?
Any comments and /simple/ references are welcome.
probability probability-theory probability-distributions manifolds smooth-manifolds
$endgroup$
I'm wondering if anyone could tell if there exists a widely accepted theory of probability distributions defined on topologically nontrivial manifolds? If so, as a physicist, I would appreciate providing some explanations using the, possibly, simplest example, a circle $S^2$.
Here are my thoughts. Generally, for a manifold $mathcal M$, I see no problem in defining some 'distribution' $f(x)$ with $xinmathcal M$, such that $int_mathcal M f(x)dmu(x)=1$. Obviously, this definition is metric-dependent. Still, oftentimes we have a canonical definition of the metric, e.g. borrowed from $mathbb R^n$ in the case if some canonical embedding is given, which is often the case.
However, we face serious difficulties when try to define 'averaged' quantities. (And in physics that's what we typically want to do).
Assume, given some 'distribution' $f(vecn)$, we want to calculate its mean value. One option would be to define it as follows:
$$
langle vec n rangle = dfracint limits_S^2 vecn f(vec n) dsleft
$$
The good thing about this definition is that it gives somewhat expected results, especially in the case of sharply-peaked distributions. However, we immediately face a huge number of problems. First of all, there exist a wide range of 'distributions' for which $langlevec nrangle$ is undefined (all the shells whose center of mass is at the origin). Second, excluding such 'bad' 'distributions' from the consideration does not really save us, for such an exclusion may be be 'quantity'-dependent (were we averaging not $vec n$ but smth else, we would have to exclude other distributions). Moreover, even if we exclude all the 'bad' ones (for a particular quantity of interest), we still cannot even define the sum for the remaining 'good' ones, for, again, the sum of 'good' distributions may be a 'bad' one.
OK, let's now consider a totally different approach suggested by the discrete probability theory. What is the mean value for the random variable which in a half cases gives $-1$, and in another half - $+1$? Well, clearly it's $0$, you would say. But wait, in terms of a 'discrete guy' who only deals with two objects in the universe, $-1$ and $+1$, this does not make any sense. There's no such object as $0$ in his universe. Nonetheless, this definition oftentimes makes sense. Why? Because we know that both $-1$ and $+1$ have a natural inclusion into $mathbb R^n$ where the mean value can be defined. Let us stop for a second and appreciate this fact - we allowed the 'mean' value of a distribution defined on the the set $mathcal S=-1,+1$ to take values on a different set $mathcalS' = [-1,1]$. (On the contrary, as of 03/2019, the canonical way of embedding heads and tails into $mathbb R^n$ is still not known, and, so, their mean value does not make much sense.)
Generalising this procedure to our example is straightforward:
$$
langle vec n rangle = int limits_S^2 vecn f(vec n) ds
$$
Which basically gives us a mean value of a distribution defined on $mathcal S'$ (again, by inclusion). An obvious downside - the averaged quantities have now no meaning for inhabitants of the $mathcal S$ manifold.
Is any of these approaches dominant? Or maybe smth else? Is there a theory for general, more complicated manifolds?
Any comments and /simple/ references are welcome.
probability probability-theory probability-distributions manifolds smooth-manifolds
probability probability-theory probability-distributions manifolds smooth-manifolds
edited Mar 2 at 6:41
mavzolej
asked Mar 2 at 6:35
mavzolejmavzolej
53628
53628
add a comment |
add a comment |
2 Answers
2
active
oldest
votes
$begingroup$
The theoretical underpinnings of probability are simple - a probability distribution is simply a (nonnegative) measure defined on some $X$ such that the total measure of $X$ is $1$. That makes perfect sense on a manifold. There's no need for any special treatment.
If it's a smooth oriented $n$-manifold, we can use that smooth structure to define the density function for a "continuous" probability distribution - that density function is a nonnegative $n$-form with integral $1$. Compact Lie groups, and compact manifolds with a transitive Lie group action, even have a standard "uniform" distribution, invariant under that Lie group action.
Now, you want to talk about expected values? We can set up those integrals $E(f)=int_mathcalM f(x),dmu(x)$ with respect to the probability measure $mu$, but only as long as the function $f$ we're trying to find the expected value of takes values in $mathbbR$, or at least some normed vector space. The expected value is a weighted sum - we need to be able to add and take scalar multiples to make sense of it at all.
That's the same normed vector space everywhere - something like a function from points on the manifold to vectors in the tangent space at those points isn't going to work (unless we embed everything into $mathbbR^m$, standardizing the tangent spaces as subspaces of that).
So then, the expected value of the position function doesn't make sense (usually). The manifold isn't a normed vector space, after all. It doesn't have an addition operation - why would we ever be able to add things up on it anyway?. On the other hand, with a particular embedding of the manifold into $mathbbR^m$, we can take an expected value of that. The uniform distribution on the sphere $S^2$, with the standard embedding into $mathbbR^3$ as $(x,y,z): x^2+y^2+z^2=1$, has an expected value of $(0,0,0)$. That's not a point on the sphere, and there was never any reason to expect it to be.
$endgroup$
$begingroup$
How would you reate this to things like Kent distribution? It does have a 'mean' vector...
$endgroup$
– mavzolej
Mar 2 at 7:22
$begingroup$
It's a probability distribution. We treat it like any other. That "mean" vector is a vector in $mathbbR^3$ coming from the standard embedding, not something in $S^2$.
$endgroup$
– jmerry
Mar 2 at 7:29
$begingroup$
So here 'mean' is just a name, it's not smth we can derive from the form of the distribution using a general formula?
$endgroup$
– mavzolej
Mar 2 at 7:31
$begingroup$
It's not just a name. We can derive it from the form of the distribution and the embedding. Once we've embedded it into $mathbbR^3$, our position vector is taking values in a normed vector space, and that's something we can take a mean of.
$endgroup$
– jmerry
Mar 2 at 7:35
$begingroup$
But the mean value in the sense of $mathbb R^n$ wouldn't belong to the sphere, it would have a smaller magnitude.
$endgroup$
– mavzolej
Mar 2 at 13:37
|
show 1 more comment
$begingroup$
One possible generalization of "mean/expectation" to metric space is called the Frechet mean/expectation which minimizes the expected value of the square distance. Let $(M, d)$ be a metric space and $X$ be a $M$-valued random variable with probability measure $P$. Then the Frechet expected value is defined as
$$
E(X) := argmin_y in M int_M d^2(X,y)dP.
$$
However the existence and uniqueness are not guaranteed. For your case, you can equip the manifold with a Riemannian metric and use the induced geodesic distance or simply use the distance induced from the embedding space.
$endgroup$
add a comment |
Your Answer
StackExchange.ready(function()
var channelOptions =
tags: "".split(" "),
id: "69"
;
initTagRenderer("".split(" "), "".split(" "), channelOptions);
StackExchange.using("externalEditor", function()
// Have to fire editor after snippets, if snippets enabled
if (StackExchange.settings.snippets.snippetsEnabled)
StackExchange.using("snippets", function()
createEditor();
);
else
createEditor();
);
function createEditor()
StackExchange.prepareEditor(
heartbeatType: 'answer',
autoActivateHeartbeat: false,
convertImagesToLinks: true,
noModals: true,
showLowRepImageUploadWarning: true,
reputationToPostImages: 10,
bindNavPrevention: true,
postfix: "",
imageUploader:
brandingHtml: "Powered by u003ca class="icon-imgur-white" href="https://imgur.com/"u003eu003c/au003e",
contentPolicyHtml: "User contributions licensed under u003ca href="https://creativecommons.org/licenses/by-sa/3.0/"u003ecc by-sa 3.0 with attribution requiredu003c/au003e u003ca href="https://stackoverflow.com/legal/content-policy"u003e(content policy)u003c/au003e",
allowUrls: true
,
noCode: true, onDemand: true,
discardSelector: ".discard-answer"
,immediatelyShowMarkdownHelp:true
);
);
Sign up or log in
StackExchange.ready(function ()
StackExchange.helpers.onClickDraftSave('#login-link');
);
Sign up using Google
Sign up using Facebook
Sign up using Email and Password
Post as a guest
Required, but never shown
StackExchange.ready(
function ()
StackExchange.openid.initPostLogin('.new-post-login', 'https%3a%2f%2fmath.stackexchange.com%2fquestions%2f3132125%2fprobability-distributions-on-topologically-nontrivial-manifolds%23new-answer', 'question_page');
);
Post as a guest
Required, but never shown
2 Answers
2
active
oldest
votes
2 Answers
2
active
oldest
votes
active
oldest
votes
active
oldest
votes
$begingroup$
The theoretical underpinnings of probability are simple - a probability distribution is simply a (nonnegative) measure defined on some $X$ such that the total measure of $X$ is $1$. That makes perfect sense on a manifold. There's no need for any special treatment.
If it's a smooth oriented $n$-manifold, we can use that smooth structure to define the density function for a "continuous" probability distribution - that density function is a nonnegative $n$-form with integral $1$. Compact Lie groups, and compact manifolds with a transitive Lie group action, even have a standard "uniform" distribution, invariant under that Lie group action.
Now, you want to talk about expected values? We can set up those integrals $E(f)=int_mathcalM f(x),dmu(x)$ with respect to the probability measure $mu$, but only as long as the function $f$ we're trying to find the expected value of takes values in $mathbbR$, or at least some normed vector space. The expected value is a weighted sum - we need to be able to add and take scalar multiples to make sense of it at all.
That's the same normed vector space everywhere - something like a function from points on the manifold to vectors in the tangent space at those points isn't going to work (unless we embed everything into $mathbbR^m$, standardizing the tangent spaces as subspaces of that).
So then, the expected value of the position function doesn't make sense (usually). The manifold isn't a normed vector space, after all. It doesn't have an addition operation - why would we ever be able to add things up on it anyway?. On the other hand, with a particular embedding of the manifold into $mathbbR^m$, we can take an expected value of that. The uniform distribution on the sphere $S^2$, with the standard embedding into $mathbbR^3$ as $(x,y,z): x^2+y^2+z^2=1$, has an expected value of $(0,0,0)$. That's not a point on the sphere, and there was never any reason to expect it to be.
$endgroup$
$begingroup$
How would you reate this to things like Kent distribution? It does have a 'mean' vector...
$endgroup$
– mavzolej
Mar 2 at 7:22
$begingroup$
It's a probability distribution. We treat it like any other. That "mean" vector is a vector in $mathbbR^3$ coming from the standard embedding, not something in $S^2$.
$endgroup$
– jmerry
Mar 2 at 7:29
$begingroup$
So here 'mean' is just a name, it's not smth we can derive from the form of the distribution using a general formula?
$endgroup$
– mavzolej
Mar 2 at 7:31
$begingroup$
It's not just a name. We can derive it from the form of the distribution and the embedding. Once we've embedded it into $mathbbR^3$, our position vector is taking values in a normed vector space, and that's something we can take a mean of.
$endgroup$
– jmerry
Mar 2 at 7:35
$begingroup$
But the mean value in the sense of $mathbb R^n$ wouldn't belong to the sphere, it would have a smaller magnitude.
$endgroup$
– mavzolej
Mar 2 at 13:37
|
show 1 more comment
$begingroup$
The theoretical underpinnings of probability are simple - a probability distribution is simply a (nonnegative) measure defined on some $X$ such that the total measure of $X$ is $1$. That makes perfect sense on a manifold. There's no need for any special treatment.
If it's a smooth oriented $n$-manifold, we can use that smooth structure to define the density function for a "continuous" probability distribution - that density function is a nonnegative $n$-form with integral $1$. Compact Lie groups, and compact manifolds with a transitive Lie group action, even have a standard "uniform" distribution, invariant under that Lie group action.
Now, you want to talk about expected values? We can set up those integrals $E(f)=int_mathcalM f(x),dmu(x)$ with respect to the probability measure $mu$, but only as long as the function $f$ we're trying to find the expected value of takes values in $mathbbR$, or at least some normed vector space. The expected value is a weighted sum - we need to be able to add and take scalar multiples to make sense of it at all.
That's the same normed vector space everywhere - something like a function from points on the manifold to vectors in the tangent space at those points isn't going to work (unless we embed everything into $mathbbR^m$, standardizing the tangent spaces as subspaces of that).
So then, the expected value of the position function doesn't make sense (usually). The manifold isn't a normed vector space, after all. It doesn't have an addition operation - why would we ever be able to add things up on it anyway?. On the other hand, with a particular embedding of the manifold into $mathbbR^m$, we can take an expected value of that. The uniform distribution on the sphere $S^2$, with the standard embedding into $mathbbR^3$ as $(x,y,z): x^2+y^2+z^2=1$, has an expected value of $(0,0,0)$. That's not a point on the sphere, and there was never any reason to expect it to be.
$endgroup$
$begingroup$
How would you reate this to things like Kent distribution? It does have a 'mean' vector...
$endgroup$
– mavzolej
Mar 2 at 7:22
$begingroup$
It's a probability distribution. We treat it like any other. That "mean" vector is a vector in $mathbbR^3$ coming from the standard embedding, not something in $S^2$.
$endgroup$
– jmerry
Mar 2 at 7:29
$begingroup$
So here 'mean' is just a name, it's not smth we can derive from the form of the distribution using a general formula?
$endgroup$
– mavzolej
Mar 2 at 7:31
$begingroup$
It's not just a name. We can derive it from the form of the distribution and the embedding. Once we've embedded it into $mathbbR^3$, our position vector is taking values in a normed vector space, and that's something we can take a mean of.
$endgroup$
– jmerry
Mar 2 at 7:35
$begingroup$
But the mean value in the sense of $mathbb R^n$ wouldn't belong to the sphere, it would have a smaller magnitude.
$endgroup$
– mavzolej
Mar 2 at 13:37
|
show 1 more comment
$begingroup$
The theoretical underpinnings of probability are simple - a probability distribution is simply a (nonnegative) measure defined on some $X$ such that the total measure of $X$ is $1$. That makes perfect sense on a manifold. There's no need for any special treatment.
If it's a smooth oriented $n$-manifold, we can use that smooth structure to define the density function for a "continuous" probability distribution - that density function is a nonnegative $n$-form with integral $1$. Compact Lie groups, and compact manifolds with a transitive Lie group action, even have a standard "uniform" distribution, invariant under that Lie group action.
Now, you want to talk about expected values? We can set up those integrals $E(f)=int_mathcalM f(x),dmu(x)$ with respect to the probability measure $mu$, but only as long as the function $f$ we're trying to find the expected value of takes values in $mathbbR$, or at least some normed vector space. The expected value is a weighted sum - we need to be able to add and take scalar multiples to make sense of it at all.
That's the same normed vector space everywhere - something like a function from points on the manifold to vectors in the tangent space at those points isn't going to work (unless we embed everything into $mathbbR^m$, standardizing the tangent spaces as subspaces of that).
So then, the expected value of the position function doesn't make sense (usually). The manifold isn't a normed vector space, after all. It doesn't have an addition operation - why would we ever be able to add things up on it anyway?. On the other hand, with a particular embedding of the manifold into $mathbbR^m$, we can take an expected value of that. The uniform distribution on the sphere $S^2$, with the standard embedding into $mathbbR^3$ as $(x,y,z): x^2+y^2+z^2=1$, has an expected value of $(0,0,0)$. That's not a point on the sphere, and there was never any reason to expect it to be.
$endgroup$
The theoretical underpinnings of probability are simple - a probability distribution is simply a (nonnegative) measure defined on some $X$ such that the total measure of $X$ is $1$. That makes perfect sense on a manifold. There's no need for any special treatment.
If it's a smooth oriented $n$-manifold, we can use that smooth structure to define the density function for a "continuous" probability distribution - that density function is a nonnegative $n$-form with integral $1$. Compact Lie groups, and compact manifolds with a transitive Lie group action, even have a standard "uniform" distribution, invariant under that Lie group action.
Now, you want to talk about expected values? We can set up those integrals $E(f)=int_mathcalM f(x),dmu(x)$ with respect to the probability measure $mu$, but only as long as the function $f$ we're trying to find the expected value of takes values in $mathbbR$, or at least some normed vector space. The expected value is a weighted sum - we need to be able to add and take scalar multiples to make sense of it at all.
That's the same normed vector space everywhere - something like a function from points on the manifold to vectors in the tangent space at those points isn't going to work (unless we embed everything into $mathbbR^m$, standardizing the tangent spaces as subspaces of that).
So then, the expected value of the position function doesn't make sense (usually). The manifold isn't a normed vector space, after all. It doesn't have an addition operation - why would we ever be able to add things up on it anyway?. On the other hand, with a particular embedding of the manifold into $mathbbR^m$, we can take an expected value of that. The uniform distribution on the sphere $S^2$, with the standard embedding into $mathbbR^3$ as $(x,y,z): x^2+y^2+z^2=1$, has an expected value of $(0,0,0)$. That's not a point on the sphere, and there was never any reason to expect it to be.
edited Mar 2 at 23:29
answered Mar 2 at 7:09


jmerryjmerry
17k11633
17k11633
$begingroup$
How would you reate this to things like Kent distribution? It does have a 'mean' vector...
$endgroup$
– mavzolej
Mar 2 at 7:22
$begingroup$
It's a probability distribution. We treat it like any other. That "mean" vector is a vector in $mathbbR^3$ coming from the standard embedding, not something in $S^2$.
$endgroup$
– jmerry
Mar 2 at 7:29
$begingroup$
So here 'mean' is just a name, it's not smth we can derive from the form of the distribution using a general formula?
$endgroup$
– mavzolej
Mar 2 at 7:31
$begingroup$
It's not just a name. We can derive it from the form of the distribution and the embedding. Once we've embedded it into $mathbbR^3$, our position vector is taking values in a normed vector space, and that's something we can take a mean of.
$endgroup$
– jmerry
Mar 2 at 7:35
$begingroup$
But the mean value in the sense of $mathbb R^n$ wouldn't belong to the sphere, it would have a smaller magnitude.
$endgroup$
– mavzolej
Mar 2 at 13:37
|
show 1 more comment
$begingroup$
How would you reate this to things like Kent distribution? It does have a 'mean' vector...
$endgroup$
– mavzolej
Mar 2 at 7:22
$begingroup$
It's a probability distribution. We treat it like any other. That "mean" vector is a vector in $mathbbR^3$ coming from the standard embedding, not something in $S^2$.
$endgroup$
– jmerry
Mar 2 at 7:29
$begingroup$
So here 'mean' is just a name, it's not smth we can derive from the form of the distribution using a general formula?
$endgroup$
– mavzolej
Mar 2 at 7:31
$begingroup$
It's not just a name. We can derive it from the form of the distribution and the embedding. Once we've embedded it into $mathbbR^3$, our position vector is taking values in a normed vector space, and that's something we can take a mean of.
$endgroup$
– jmerry
Mar 2 at 7:35
$begingroup$
But the mean value in the sense of $mathbb R^n$ wouldn't belong to the sphere, it would have a smaller magnitude.
$endgroup$
– mavzolej
Mar 2 at 13:37
$begingroup$
How would you reate this to things like Kent distribution? It does have a 'mean' vector...
$endgroup$
– mavzolej
Mar 2 at 7:22
$begingroup$
How would you reate this to things like Kent distribution? It does have a 'mean' vector...
$endgroup$
– mavzolej
Mar 2 at 7:22
$begingroup$
It's a probability distribution. We treat it like any other. That "mean" vector is a vector in $mathbbR^3$ coming from the standard embedding, not something in $S^2$.
$endgroup$
– jmerry
Mar 2 at 7:29
$begingroup$
It's a probability distribution. We treat it like any other. That "mean" vector is a vector in $mathbbR^3$ coming from the standard embedding, not something in $S^2$.
$endgroup$
– jmerry
Mar 2 at 7:29
$begingroup$
So here 'mean' is just a name, it's not smth we can derive from the form of the distribution using a general formula?
$endgroup$
– mavzolej
Mar 2 at 7:31
$begingroup$
So here 'mean' is just a name, it's not smth we can derive from the form of the distribution using a general formula?
$endgroup$
– mavzolej
Mar 2 at 7:31
$begingroup$
It's not just a name. We can derive it from the form of the distribution and the embedding. Once we've embedded it into $mathbbR^3$, our position vector is taking values in a normed vector space, and that's something we can take a mean of.
$endgroup$
– jmerry
Mar 2 at 7:35
$begingroup$
It's not just a name. We can derive it from the form of the distribution and the embedding. Once we've embedded it into $mathbbR^3$, our position vector is taking values in a normed vector space, and that's something we can take a mean of.
$endgroup$
– jmerry
Mar 2 at 7:35
$begingroup$
But the mean value in the sense of $mathbb R^n$ wouldn't belong to the sphere, it would have a smaller magnitude.
$endgroup$
– mavzolej
Mar 2 at 13:37
$begingroup$
But the mean value in the sense of $mathbb R^n$ wouldn't belong to the sphere, it would have a smaller magnitude.
$endgroup$
– mavzolej
Mar 2 at 13:37
|
show 1 more comment
$begingroup$
One possible generalization of "mean/expectation" to metric space is called the Frechet mean/expectation which minimizes the expected value of the square distance. Let $(M, d)$ be a metric space and $X$ be a $M$-valued random variable with probability measure $P$. Then the Frechet expected value is defined as
$$
E(X) := argmin_y in M int_M d^2(X,y)dP.
$$
However the existence and uniqueness are not guaranteed. For your case, you can equip the manifold with a Riemannian metric and use the induced geodesic distance or simply use the distance induced from the embedding space.
$endgroup$
add a comment |
$begingroup$
One possible generalization of "mean/expectation" to metric space is called the Frechet mean/expectation which minimizes the expected value of the square distance. Let $(M, d)$ be a metric space and $X$ be a $M$-valued random variable with probability measure $P$. Then the Frechet expected value is defined as
$$
E(X) := argmin_y in M int_M d^2(X,y)dP.
$$
However the existence and uniqueness are not guaranteed. For your case, you can equip the manifold with a Riemannian metric and use the induced geodesic distance or simply use the distance induced from the embedding space.
$endgroup$
add a comment |
$begingroup$
One possible generalization of "mean/expectation" to metric space is called the Frechet mean/expectation which minimizes the expected value of the square distance. Let $(M, d)$ be a metric space and $X$ be a $M$-valued random variable with probability measure $P$. Then the Frechet expected value is defined as
$$
E(X) := argmin_y in M int_M d^2(X,y)dP.
$$
However the existence and uniqueness are not guaranteed. For your case, you can equip the manifold with a Riemannian metric and use the induced geodesic distance or simply use the distance induced from the embedding space.
$endgroup$
One possible generalization of "mean/expectation" to metric space is called the Frechet mean/expectation which minimizes the expected value of the square distance. Let $(M, d)$ be a metric space and $X$ be a $M$-valued random variable with probability measure $P$. Then the Frechet expected value is defined as
$$
E(X) := argmin_y in M int_M d^2(X,y)dP.
$$
However the existence and uniqueness are not guaranteed. For your case, you can equip the manifold with a Riemannian metric and use the induced geodesic distance or simply use the distance induced from the embedding space.
answered Apr 1 at 13:58
chunhaochunhao
61
61
add a comment |
add a comment |
Thanks for contributing an answer to Mathematics Stack Exchange!
- Please be sure to answer the question. Provide details and share your research!
But avoid …
- Asking for help, clarification, or responding to other answers.
- Making statements based on opinion; back them up with references or personal experience.
Use MathJax to format equations. MathJax reference.
To learn more, see our tips on writing great answers.
Sign up or log in
StackExchange.ready(function ()
StackExchange.helpers.onClickDraftSave('#login-link');
);
Sign up using Google
Sign up using Facebook
Sign up using Email and Password
Post as a guest
Required, but never shown
StackExchange.ready(
function ()
StackExchange.openid.initPostLogin('.new-post-login', 'https%3a%2f%2fmath.stackexchange.com%2fquestions%2f3132125%2fprobability-distributions-on-topologically-nontrivial-manifolds%23new-answer', 'question_page');
);
Post as a guest
Required, but never shown
Sign up or log in
StackExchange.ready(function ()
StackExchange.helpers.onClickDraftSave('#login-link');
);
Sign up using Google
Sign up using Facebook
Sign up using Email and Password
Post as a guest
Required, but never shown
Sign up or log in
StackExchange.ready(function ()
StackExchange.helpers.onClickDraftSave('#login-link');
);
Sign up using Google
Sign up using Facebook
Sign up using Email and Password
Post as a guest
Required, but never shown
Sign up or log in
StackExchange.ready(function ()
StackExchange.helpers.onClickDraftSave('#login-link');
);
Sign up using Google
Sign up using Facebook
Sign up using Email and Password
Sign up using Google
Sign up using Facebook
Sign up using Email and Password
Post as a guest
Required, but never shown
Required, but never shown
Required, but never shown
Required, but never shown
Required, but never shown
Required, but never shown
Required, but never shown
Required, but never shown
Required, but never shown
dwDF,hotCec6,1 YYaLa E4s4jJ 9D4NFzE6Vkn,j0