For a Maximum Likelihood Estimation with events that implicate each other, how should the likelihood function be constructed? The Next CEO of Stack OverflowMaximum Likelihood (ML) vs Maximum a Posteriori Estimation (MAP), When to Use Which?Maximum Likelihood Estimation with Indicator FunctionLikelihood Function is a random variableProof for Maximum Likelihood EstimationDerivative of expected log likelihood in a logistic regression modelMaximum likelihood for the Brownian Motion with driftHow to prove that the maximum likelihood estimator of θ is aysmptotically unbiased and cosistent for a general density functionAs n approaches infinity, the likelihood function at true parameter values is maximum among any other feasible choice of the parametersCan someone recommend a book that covers (and proofs) asymptotic properties of Maximum Likelihood Estimation?Maximum likelihood estimation for a subset of data and split model
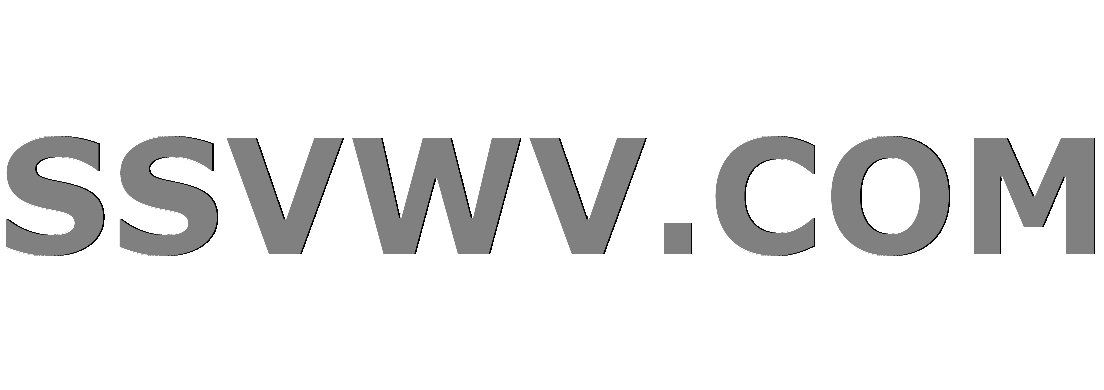
Multi tool use
When did Lisp start using symbols for arithmetic?
Is it okay to store user locations?
Is it my responsibility to learn a new technology in my own time my employer wants to implement?
Can the Reverse Gravity spell affect the Meteor Swarm spell?
Does the Brexit deal have to be agreed by both Houses?
Go Pregnant or Go Home
How can I quit an app using Terminal?
When airplanes disconnect from a tanker during air to air refueling, why do they bank so sharply to the right?
Can I equip Skullclamp on a creature I am sacrificing?
I believe this to be a fraud - hired, then asked to cash check and send cash as Bitcoin
Why is there a PLL in CPU?
Unreliable Magic - Is it worth it?
If the heap is initialized for security, then why is the stack uninitialized?
How to get regions to plot as graphics
Is it a good idea to use COLUMN AS (left([Another_Column],(4)) instead of LEFT in the select?
Why didn't Khan get resurrected in the Genesis Explosion?
Would this house-rule that treats advantage as a +1 to the roll instead (and disadvantage as -1) and allows them to stack be balanced?
What does this shorthand mean?
How to Reset Passwords on Multiple Websites Easily?
How to write papers efficiently when English isn't my first language?
Term for the "extreme-extension" version of a straw man fallacy?
Only print output after finding pattern
How do I construct this japanese bowl?
Rotate a column
For a Maximum Likelihood Estimation with events that implicate each other, how should the likelihood function be constructed?
The Next CEO of Stack OverflowMaximum Likelihood (ML) vs Maximum a Posteriori Estimation (MAP), When to Use Which?Maximum Likelihood Estimation with Indicator FunctionLikelihood Function is a random variableProof for Maximum Likelihood EstimationDerivative of expected log likelihood in a logistic regression modelMaximum likelihood for the Brownian Motion with driftHow to prove that the maximum likelihood estimator of θ is aysmptotically unbiased and cosistent for a general density functionAs n approaches infinity, the likelihood function at true parameter values is maximum among any other feasible choice of the parametersCan someone recommend a book that covers (and proofs) asymptotic properties of Maximum Likelihood Estimation?Maximum likelihood estimation for a subset of data and split model
$begingroup$
The probability of a student with a skill parameter of "s" to obtain at least a score of "k" in a certain test is defined as:
$$frac1e^b_k-s+1$$
Where $b_k$ is a difficulty parameter of achieving at least that score ($b_0$ is $-infty$ since the student always gets at least 0 points). The maximum score in the test is m. In the data there are several tests (each with their own difficulty parameters, all with the same value of m), and several students (each with their own skill parameter).
For example, if the student achieves a score of 2 out of 5, that implies that they got at least 2 points but failed to get at least 3 points, so the likelihood is:
$$frac1e^b_2-s+1-frac1e^b_3-s+1$$
(Getting at least 3 points implies getting more than 2 points, so the likelihood of achieving 2 points is the difference in likelihood between getting at least 2 points and at least 3 points)
But using that likelihood function for maximum likelihood estimation gives odd results for the difficulty parameters with fixed student skill parameters (a higher score doesn't always imply an equal or higher difficulty, or sometimes some difficulty parameters are undetermined), instead, using a likelihood function of:
$$fracleft(1-frac1e^b_3-s+1right) left(1-frac1e^b_4-s+1right) left(1-frac1e^b_5-s+1right)left(e^b_1-s+1right) left(e^b_2-s+1right)$$
(This treats a single score as 5 separate events, achieving at least 1 and 2 points, but failing to achieve at least 3, 4 and 5 points)
Seems to work better for difficulty parameter estimation.
On the other hand, when estimating the skill parameters of the students, the first likelihood function seems to work better compared to the second one (the values obtained are too high for students that score perfectly in one of the tests but does mediocre on the others, compared to students that consistently score almost perfectly).
Since both the skill and difficulty parameters have to be estimated, it seems odd to me that one likelihood function works better for some parameters but not the others. Also there is the question of which function is better justified mathematically (maybe I'm doing something wrong, and the proper likelihood function is something else entirely).
maximum-likelihood logistic-regression
New contributor
Dropped Bass is a new contributor to this site. Take care in asking for clarification, commenting, and answering.
Check out our Code of Conduct.
$endgroup$
add a comment |
$begingroup$
The probability of a student with a skill parameter of "s" to obtain at least a score of "k" in a certain test is defined as:
$$frac1e^b_k-s+1$$
Where $b_k$ is a difficulty parameter of achieving at least that score ($b_0$ is $-infty$ since the student always gets at least 0 points). The maximum score in the test is m. In the data there are several tests (each with their own difficulty parameters, all with the same value of m), and several students (each with their own skill parameter).
For example, if the student achieves a score of 2 out of 5, that implies that they got at least 2 points but failed to get at least 3 points, so the likelihood is:
$$frac1e^b_2-s+1-frac1e^b_3-s+1$$
(Getting at least 3 points implies getting more than 2 points, so the likelihood of achieving 2 points is the difference in likelihood between getting at least 2 points and at least 3 points)
But using that likelihood function for maximum likelihood estimation gives odd results for the difficulty parameters with fixed student skill parameters (a higher score doesn't always imply an equal or higher difficulty, or sometimes some difficulty parameters are undetermined), instead, using a likelihood function of:
$$fracleft(1-frac1e^b_3-s+1right) left(1-frac1e^b_4-s+1right) left(1-frac1e^b_5-s+1right)left(e^b_1-s+1right) left(e^b_2-s+1right)$$
(This treats a single score as 5 separate events, achieving at least 1 and 2 points, but failing to achieve at least 3, 4 and 5 points)
Seems to work better for difficulty parameter estimation.
On the other hand, when estimating the skill parameters of the students, the first likelihood function seems to work better compared to the second one (the values obtained are too high for students that score perfectly in one of the tests but does mediocre on the others, compared to students that consistently score almost perfectly).
Since both the skill and difficulty parameters have to be estimated, it seems odd to me that one likelihood function works better for some parameters but not the others. Also there is the question of which function is better justified mathematically (maybe I'm doing something wrong, and the proper likelihood function is something else entirely).
maximum-likelihood logistic-regression
New contributor
Dropped Bass is a new contributor to this site. Take care in asking for clarification, commenting, and answering.
Check out our Code of Conduct.
$endgroup$
add a comment |
$begingroup$
The probability of a student with a skill parameter of "s" to obtain at least a score of "k" in a certain test is defined as:
$$frac1e^b_k-s+1$$
Where $b_k$ is a difficulty parameter of achieving at least that score ($b_0$ is $-infty$ since the student always gets at least 0 points). The maximum score in the test is m. In the data there are several tests (each with their own difficulty parameters, all with the same value of m), and several students (each with their own skill parameter).
For example, if the student achieves a score of 2 out of 5, that implies that they got at least 2 points but failed to get at least 3 points, so the likelihood is:
$$frac1e^b_2-s+1-frac1e^b_3-s+1$$
(Getting at least 3 points implies getting more than 2 points, so the likelihood of achieving 2 points is the difference in likelihood between getting at least 2 points and at least 3 points)
But using that likelihood function for maximum likelihood estimation gives odd results for the difficulty parameters with fixed student skill parameters (a higher score doesn't always imply an equal or higher difficulty, or sometimes some difficulty parameters are undetermined), instead, using a likelihood function of:
$$fracleft(1-frac1e^b_3-s+1right) left(1-frac1e^b_4-s+1right) left(1-frac1e^b_5-s+1right)left(e^b_1-s+1right) left(e^b_2-s+1right)$$
(This treats a single score as 5 separate events, achieving at least 1 and 2 points, but failing to achieve at least 3, 4 and 5 points)
Seems to work better for difficulty parameter estimation.
On the other hand, when estimating the skill parameters of the students, the first likelihood function seems to work better compared to the second one (the values obtained are too high for students that score perfectly in one of the tests but does mediocre on the others, compared to students that consistently score almost perfectly).
Since both the skill and difficulty parameters have to be estimated, it seems odd to me that one likelihood function works better for some parameters but not the others. Also there is the question of which function is better justified mathematically (maybe I'm doing something wrong, and the proper likelihood function is something else entirely).
maximum-likelihood logistic-regression
New contributor
Dropped Bass is a new contributor to this site. Take care in asking for clarification, commenting, and answering.
Check out our Code of Conduct.
$endgroup$
The probability of a student with a skill parameter of "s" to obtain at least a score of "k" in a certain test is defined as:
$$frac1e^b_k-s+1$$
Where $b_k$ is a difficulty parameter of achieving at least that score ($b_0$ is $-infty$ since the student always gets at least 0 points). The maximum score in the test is m. In the data there are several tests (each with their own difficulty parameters, all with the same value of m), and several students (each with their own skill parameter).
For example, if the student achieves a score of 2 out of 5, that implies that they got at least 2 points but failed to get at least 3 points, so the likelihood is:
$$frac1e^b_2-s+1-frac1e^b_3-s+1$$
(Getting at least 3 points implies getting more than 2 points, so the likelihood of achieving 2 points is the difference in likelihood between getting at least 2 points and at least 3 points)
But using that likelihood function for maximum likelihood estimation gives odd results for the difficulty parameters with fixed student skill parameters (a higher score doesn't always imply an equal or higher difficulty, or sometimes some difficulty parameters are undetermined), instead, using a likelihood function of:
$$fracleft(1-frac1e^b_3-s+1right) left(1-frac1e^b_4-s+1right) left(1-frac1e^b_5-s+1right)left(e^b_1-s+1right) left(e^b_2-s+1right)$$
(This treats a single score as 5 separate events, achieving at least 1 and 2 points, but failing to achieve at least 3, 4 and 5 points)
Seems to work better for difficulty parameter estimation.
On the other hand, when estimating the skill parameters of the students, the first likelihood function seems to work better compared to the second one (the values obtained are too high for students that score perfectly in one of the tests but does mediocre on the others, compared to students that consistently score almost perfectly).
Since both the skill and difficulty parameters have to be estimated, it seems odd to me that one likelihood function works better for some parameters but not the others. Also there is the question of which function is better justified mathematically (maybe I'm doing something wrong, and the proper likelihood function is something else entirely).
maximum-likelihood logistic-regression
maximum-likelihood logistic-regression
New contributor
Dropped Bass is a new contributor to this site. Take care in asking for clarification, commenting, and answering.
Check out our Code of Conduct.
New contributor
Dropped Bass is a new contributor to this site. Take care in asking for clarification, commenting, and answering.
Check out our Code of Conduct.
edited yesterday
J. W. Tanner
4,0171320
4,0171320
New contributor
Dropped Bass is a new contributor to this site. Take care in asking for clarification, commenting, and answering.
Check out our Code of Conduct.
asked yesterday


Dropped BassDropped Bass
12
12
New contributor
Dropped Bass is a new contributor to this site. Take care in asking for clarification, commenting, and answering.
Check out our Code of Conduct.
New contributor
Dropped Bass is a new contributor to this site. Take care in asking for clarification, commenting, and answering.
Check out our Code of Conduct.
Dropped Bass is a new contributor to this site. Take care in asking for clarification, commenting, and answering.
Check out our Code of Conduct.
add a comment |
add a comment |
0
active
oldest
votes
Your Answer
StackExchange.ifUsing("editor", function ()
return StackExchange.using("mathjaxEditing", function ()
StackExchange.MarkdownEditor.creationCallbacks.add(function (editor, postfix)
StackExchange.mathjaxEditing.prepareWmdForMathJax(editor, postfix, [["$", "$"], ["\\(","\\)"]]);
);
);
, "mathjax-editing");
StackExchange.ready(function()
var channelOptions =
tags: "".split(" "),
id: "69"
;
initTagRenderer("".split(" "), "".split(" "), channelOptions);
StackExchange.using("externalEditor", function()
// Have to fire editor after snippets, if snippets enabled
if (StackExchange.settings.snippets.snippetsEnabled)
StackExchange.using("snippets", function()
createEditor();
);
else
createEditor();
);
function createEditor()
StackExchange.prepareEditor(
heartbeatType: 'answer',
autoActivateHeartbeat: false,
convertImagesToLinks: true,
noModals: true,
showLowRepImageUploadWarning: true,
reputationToPostImages: 10,
bindNavPrevention: true,
postfix: "",
imageUploader:
brandingHtml: "Powered by u003ca class="icon-imgur-white" href="https://imgur.com/"u003eu003c/au003e",
contentPolicyHtml: "User contributions licensed under u003ca href="https://creativecommons.org/licenses/by-sa/3.0/"u003ecc by-sa 3.0 with attribution requiredu003c/au003e u003ca href="https://stackoverflow.com/legal/content-policy"u003e(content policy)u003c/au003e",
allowUrls: true
,
noCode: true, onDemand: true,
discardSelector: ".discard-answer"
,immediatelyShowMarkdownHelp:true
);
);
Dropped Bass is a new contributor. Be nice, and check out our Code of Conduct.
Sign up or log in
StackExchange.ready(function ()
StackExchange.helpers.onClickDraftSave('#login-link');
);
Sign up using Google
Sign up using Facebook
Sign up using Email and Password
Post as a guest
Required, but never shown
StackExchange.ready(
function ()
StackExchange.openid.initPostLogin('.new-post-login', 'https%3a%2f%2fmath.stackexchange.com%2fquestions%2f3164367%2ffor-a-maximum-likelihood-estimation-with-events-that-implicate-each-other-how-s%23new-answer', 'question_page');
);
Post as a guest
Required, but never shown
0
active
oldest
votes
0
active
oldest
votes
active
oldest
votes
active
oldest
votes
Dropped Bass is a new contributor. Be nice, and check out our Code of Conduct.
Dropped Bass is a new contributor. Be nice, and check out our Code of Conduct.
Dropped Bass is a new contributor. Be nice, and check out our Code of Conduct.
Dropped Bass is a new contributor. Be nice, and check out our Code of Conduct.
Thanks for contributing an answer to Mathematics Stack Exchange!
- Please be sure to answer the question. Provide details and share your research!
But avoid …
- Asking for help, clarification, or responding to other answers.
- Making statements based on opinion; back them up with references or personal experience.
Use MathJax to format equations. MathJax reference.
To learn more, see our tips on writing great answers.
Sign up or log in
StackExchange.ready(function ()
StackExchange.helpers.onClickDraftSave('#login-link');
);
Sign up using Google
Sign up using Facebook
Sign up using Email and Password
Post as a guest
Required, but never shown
StackExchange.ready(
function ()
StackExchange.openid.initPostLogin('.new-post-login', 'https%3a%2f%2fmath.stackexchange.com%2fquestions%2f3164367%2ffor-a-maximum-likelihood-estimation-with-events-that-implicate-each-other-how-s%23new-answer', 'question_page');
);
Post as a guest
Required, but never shown
Sign up or log in
StackExchange.ready(function ()
StackExchange.helpers.onClickDraftSave('#login-link');
);
Sign up using Google
Sign up using Facebook
Sign up using Email and Password
Post as a guest
Required, but never shown
Sign up or log in
StackExchange.ready(function ()
StackExchange.helpers.onClickDraftSave('#login-link');
);
Sign up using Google
Sign up using Facebook
Sign up using Email and Password
Post as a guest
Required, but never shown
Sign up or log in
StackExchange.ready(function ()
StackExchange.helpers.onClickDraftSave('#login-link');
);
Sign up using Google
Sign up using Facebook
Sign up using Email and Password
Sign up using Google
Sign up using Facebook
Sign up using Email and Password
Post as a guest
Required, but never shown
Required, but never shown
Required, but never shown
Required, but never shown
Required, but never shown
Required, but never shown
Required, but never shown
Required, but never shown
Required, but never shown
sLhng6 v3kyiRPGMLs2AJ4rTYU,JWpkZustvI i6wCXL,BqaBWh,712X,iuBL3kE1c,TqRVT4XtcBLzAjL58TPJE