Regression vs Random Forest - Combination of features Announcing the arrival of Valued Associate #679: Cesar Manara Planned maintenance scheduled April 17/18, 2019 at 00:00UTC (8:00pm US/Eastern) 2019 Moderator Election Q&A - Questionnaire 2019 Community Moderator Election ResultsHow important is lookahead search in decision trees?feature importance via random forest and linear regression are differentsklearn random forest and fitting with continuous featuresWhy do we pick random features in random forestMultiple time-series predictions with Random Forests (in Python)Forecast Model recognize future trendFeatures selection/combination for random forestGet frequent features of scikitlearn random forestMetrics to evaluate features' importance in classification problem (with random forest)Mean Absolute Error in Random Forest Regression
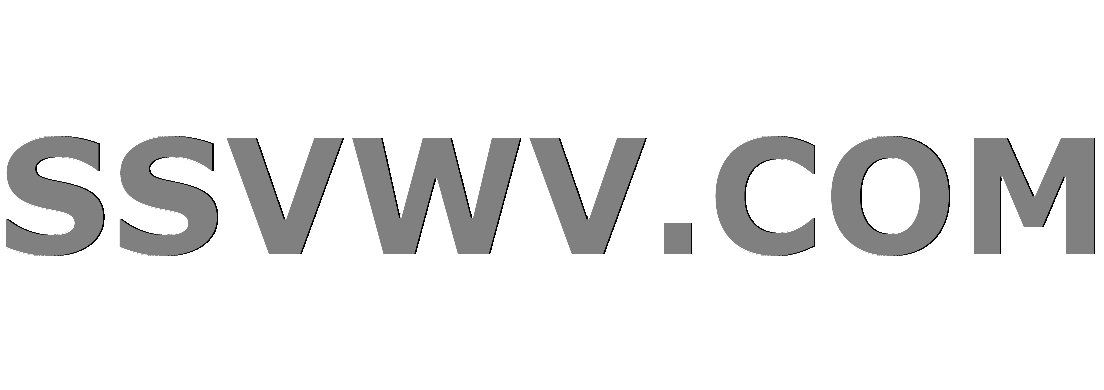
Multi tool use
When communicating altitude with a '9' in it, should it be pronounced "nine hundred" or "niner hundred"?
Fishing simulator
Stop battery usage [Ubuntu 18]
grandmas drink with lemon juice
How do you clear the ApexPages.getMessages() collection in a test?
Stars Make Stars
How can you insert a "times/divide" symbol similar to the "plus/minus" (±) one?
Is there a documented rationale why the House Ways and Means chairman can demand tax info?
3 doors, three guards, one stone
What was the last x86 CPU that did not have the x87 floating-point unit built in?
Is it possible to ask for a hotel room without minibar/extra services?
I'm having difficulty getting my players to do stuff in a sandbox campaign
How to set letter above or below the symbol?
Why does tar appear to skip file contents when output file is /dev/null?
Determine whether or not the following series converge.
How can I make names more distinctive without making them longer?
New Order #5: where Fibonacci and Beatty meet at Wythoff
Slither Like a Snake
Can a zero nonce be safely used with AES-GCM if the key is random and never used again?
Who can trigger ship-wide alerts in Star Trek?
What LEGO pieces have "real-world" functionality?
What's the point in a preamp?
Classification of bundles, Postnikov towers, obstruction theory, local coefficients
How do I keep my slimes from escaping their pens?
Regression vs Random Forest - Combination of features
Announcing the arrival of Valued Associate #679: Cesar Manara
Planned maintenance scheduled April 17/18, 2019 at 00:00UTC (8:00pm US/Eastern)
2019 Moderator Election Q&A - Questionnaire
2019 Community Moderator Election ResultsHow important is lookahead search in decision trees?feature importance via random forest and linear regression are differentsklearn random forest and fitting with continuous featuresWhy do we pick random features in random forestMultiple time-series predictions with Random Forests (in Python)Forecast Model recognize future trendFeatures selection/combination for random forestGet frequent features of scikitlearn random forestMetrics to evaluate features' importance in classification problem (with random forest)Mean Absolute Error in Random Forest Regression
$begingroup$
I had a discussion with a friend and we were talking about the advantages of random forest over linear regression.
At some point, my friend said that one of the advantages of the random forest over the linear regression is that it takes automatically into account the combination of features.
By this he meant that if I have a model with
- Y as a target
- X, W, Z as the predictors
then the random forests tests also the combinations of the features (e.g. X+W) whereas in linear regression you have to build these manually and insert them at the model.
I am quite confused, is this true?
Also if it true then is it about any kind of combination of features (e.g. X*W, X+W+Z etc) or only for some specific ones (e.g. X+W)?
feature-selection random-forest feature-engineering
$endgroup$
add a comment |
$begingroup$
I had a discussion with a friend and we were talking about the advantages of random forest over linear regression.
At some point, my friend said that one of the advantages of the random forest over the linear regression is that it takes automatically into account the combination of features.
By this he meant that if I have a model with
- Y as a target
- X, W, Z as the predictors
then the random forests tests also the combinations of the features (e.g. X+W) whereas in linear regression you have to build these manually and insert them at the model.
I am quite confused, is this true?
Also if it true then is it about any kind of combination of features (e.g. X*W, X+W+Z etc) or only for some specific ones (e.g. X+W)?
feature-selection random-forest feature-engineering
$endgroup$
add a comment |
$begingroup$
I had a discussion with a friend and we were talking about the advantages of random forest over linear regression.
At some point, my friend said that one of the advantages of the random forest over the linear regression is that it takes automatically into account the combination of features.
By this he meant that if I have a model with
- Y as a target
- X, W, Z as the predictors
then the random forests tests also the combinations of the features (e.g. X+W) whereas in linear regression you have to build these manually and insert them at the model.
I am quite confused, is this true?
Also if it true then is it about any kind of combination of features (e.g. X*W, X+W+Z etc) or only for some specific ones (e.g. X+W)?
feature-selection random-forest feature-engineering
$endgroup$
I had a discussion with a friend and we were talking about the advantages of random forest over linear regression.
At some point, my friend said that one of the advantages of the random forest over the linear regression is that it takes automatically into account the combination of features.
By this he meant that if I have a model with
- Y as a target
- X, W, Z as the predictors
then the random forests tests also the combinations of the features (e.g. X+W) whereas in linear regression you have to build these manually and insert them at the model.
I am quite confused, is this true?
Also if it true then is it about any kind of combination of features (e.g. X*W, X+W+Z etc) or only for some specific ones (e.g. X+W)?
feature-selection random-forest feature-engineering
feature-selection random-forest feature-engineering
edited Mar 31 at 22:07
Poete Maudit
asked Mar 31 at 14:28
Poete MauditPoete Maudit
426315
426315
add a comment |
add a comment |
3 Answers
3
active
oldest
votes
$begingroup$
I think it is true. Tree based algorithms especially the ones with multiple trees has the capability of capturing different feature interactions. Please see this article from xgboost official documentation and this discussion. You can say it's a perk of being a non parametric model (trees are non parametric and linear regression is not). I hope this will shed some light on this thought.
$endgroup$
$begingroup$
(+1) As an example,Tree 1 works with features (A, B) and gives 80% accuracy, Tree 2 works with features (C, D) and gives 60%. A boosting algorithm puts more weight on Tree 1, thus effectively favors f(A, B) over g(C, D).
$endgroup$
– Esmailian
Mar 31 at 19:14
$begingroup$
Thank you for your answer. However, to be honest I would like a more in depth answer. To start with, my second question is still unanswered I think: "Also if it true then is it about any kind of combination of features (e.g. X*W, X+W+Z etc) or only for some specific ones (e.g. X+W)?"
$endgroup$
– Poete Maudit
Apr 1 at 10:49
$begingroup$
Please refer this link ( mariofilho.com/can-gradient-boosting-learn-simple-arithmetic ) . This article talks about how boosting trees can model arithmetic operations like X*W, X/W, etc. Theoretically, it is possible. Trees are like neural networks, they are universal approximator (Theoretically). And I am stressing on the word Theoretically.
$endgroup$
– tam
Apr 1 at 11:05
$begingroup$
Ok thank you for this too. However, to start with both the other people here are claiming the opposite than you so it is quite difficult for me to draw a definite conclusion.
$endgroup$
– Poete Maudit
Apr 1 at 11:26
$begingroup$
Also by the way at your answer you are saying "... has the capability of capturing different feature interactions". However, my question is whether is built-in in random forest (or in boosting algos). In a sense, linear regression also has the "capability" of doing this but exactly you will have to programme it i.e. add some lines of code where you are adding, multiplying some of the features etc.
$endgroup$
– Poete Maudit
Apr 1 at 14:04
|
show 1 more comment
$begingroup$
I would say it is not true as Random forests which are made up of decision trees does perform feature selection but they do not perform feature engineering (feature selection is different from feature engineering). Decision trees use a metric called Information gain (which is total entropy minus the weighted entropy) as per which useful features are separated from bad features. Simply to say whichever feature exhibit the highest information gain on this iteration is chosen as the node on which the tree on this iteration is split or you can say which feature reduces the entropy(aka randomness) the most in this iteration is chosen as the node upon which the tree is split on this iteration. So if you data is text, trees are split upon words. If your data is real valued numbers, tree is split upon that. Hope it helps
For more details check this
$endgroup$
$begingroup$
Thank you for your answer. However, to be honest I would like a more in depth answer. To start with, my second question is still unanswered I think: "Also if it true then is it about any kind of combination of features (e.g. X*W, X+W+Z etc) or only for some specific ones (e.g. X+W)?"
$endgroup$
– Poete Maudit
Apr 1 at 10:49
$begingroup$
Yes as said in my previous answer, decision trees cannot perform feature engineering by themselves. They pick the right feature based on information gain which is called as the feature selection. So (X+W), (X*W) or any sort of simple or complex feature engineered features are not possible in case of tree based models. So answer to your second question is "No, Tree based methods cannot and will not perform feature engineering on their own". Hope it's clear
$endgroup$
– karthikeyan mg
Apr 1 at 11:15
$begingroup$
Now it is significantly clearer because your starting phrase "I would say it is partly true as Random forests..." confuses things a bit. So basically at my question your answer is "no it is not true; random forest does not take into account the combination of features e.g. X+W etc". It would be good to modify a bit your post because this is not evident.
$endgroup$
– Poete Maudit
Apr 1 at 11:23
$begingroup$
However, I will have to see some evidence on why the boosting algorithms do this while the bagging algorithms do not. Also, in the case of the boosting algorithms how the algorithm chooses which of the various combinations to test?
$endgroup$
– Poete Maudit
Apr 1 at 11:25
$begingroup$
Thanks for the suggestion, I've made the changes. And regarding your last comment, just to be clear, random forests comes under bagging algos and gbdt, xgboost comes under boosting. I'd suggest you draft another question explaining your last comment in detail along with your thoughts and understanding and link the question here, We will try our best to help you! Cheers
$endgroup$
– karthikeyan mg
Apr 1 at 11:48
|
show 1 more comment
$begingroup$
The statement "it tests combination of features" is not true. It tests individual features. However, a tree can approximate any continuous function $f$ over training points, since it is a universal approximator just like neural networks.
In Random Forest (or Decision Tree, or Regression Tree), individual features are compared to each other, not a combination of them, then the most informative individual is peaked to split a leaf. Therefore, there is no notion of "better combination" in the whole process.
Furthermore, Random Forest is a bagging algorithm which does not favor the randomly-built trees over each other, they all have the same weight in the aggregated output.
It is worth noting that "Rotation forest" first applies PCA to features, which means each new feature is a linear combination of original features. However, this does not count since the same pre-processing can be used for any other method too.
EDIT:
@tam provided a counter-example for XGBoost, which is not the same as Random Forest. However, the issue is the same for XGBoost. Its learning process comes down to splitting each leaf greadily based on a single feature instead of selecting the best combination of features among a set of combinations, or the best tree among a set of trees.
From this explanation, you can see that The Structure Score is defined for a tree (which is a function) based on the first- and second-order derivatives of loss function in each leaf $j$ ($G_j$ and $H_j$ respectively) summed over all $T$ leaves, i.e.
$$textobj^*=-frac12 sum_j=1^TfracG_jH_j + lambda + gamma T$$
However, the optimization process greedily splits a leaf using the best individual feature that gives the highest gain in $textobj^*$.
A tree $t$ is built by greedily minimizing the loss, i.e. branching on the best individual feature, and when the tree is built, process goes to create the next tree $t+1$ in the same way, and so on.
Here is the key quote from XGBoost paper:
This score is like the impurity score for evaluating decision trees,
except that it is derived for a wider range of objective functions [..] Normally it is impossible to enumerate all the possible tree
structures q. A greedy algorithm that starts from a single leaf and
iteratively adds branches to the tree is used instead.
In summary:
Although a tree represents a combination of features (a function), but
none of XGBoost and Random Forest are selecting between functions.
They build and aggregate multiple functions by greedily favoring individual
features.
$endgroup$
$begingroup$
Thank you for your answer. My post triggered some opposing views and now in this sense I do not know yet which side to take. By the way, my impression is that the remark of @tam is not really directly to the point. The fact that tree boosting algorithms favor f(X, Y) over g(Y, W) does not necessarily mean that they take into account the combination of the features in the sense of e.g. X+W but they simply favor groups of features over other groups of features. Thus, not combination of features but groups of features (if I am not missing anything).
$endgroup$
– Poete Maudit
Apr 1 at 10:56
$begingroup$
@PoeteMaudit I added an example.
$endgroup$
– Esmailian
Apr 1 at 11:04
$begingroup$
Cool, thank you. However, I will have to see some evidence on why the boosting algorithms do this while the bagging algorithms do not. Also, in the case of the boosting algorithms how the algorithm chooses which of the various combinations to test?
$endgroup$
– Poete Maudit
Apr 1 at 11:25
1
$begingroup$
So your answer to my question is that "Note that, a tree can approximate any continuous function f over training points, since it is a universal approximator just like neural networks."? If so then this is interesting.
$endgroup$
– Poete Maudit
Apr 1 at 13:55
add a comment |
Your Answer
StackExchange.ready(function()
var channelOptions =
tags: "".split(" "),
id: "557"
;
initTagRenderer("".split(" "), "".split(" "), channelOptions);
StackExchange.using("externalEditor", function()
// Have to fire editor after snippets, if snippets enabled
if (StackExchange.settings.snippets.snippetsEnabled)
StackExchange.using("snippets", function()
createEditor();
);
else
createEditor();
);
function createEditor()
StackExchange.prepareEditor(
heartbeatType: 'answer',
autoActivateHeartbeat: false,
convertImagesToLinks: false,
noModals: true,
showLowRepImageUploadWarning: true,
reputationToPostImages: null,
bindNavPrevention: true,
postfix: "",
imageUploader:
brandingHtml: "Powered by u003ca class="icon-imgur-white" href="https://imgur.com/"u003eu003c/au003e",
contentPolicyHtml: "User contributions licensed under u003ca href="https://creativecommons.org/licenses/by-sa/3.0/"u003ecc by-sa 3.0 with attribution requiredu003c/au003e u003ca href="https://stackoverflow.com/legal/content-policy"u003e(content policy)u003c/au003e",
allowUrls: true
,
onDemand: true,
discardSelector: ".discard-answer"
,immediatelyShowMarkdownHelp:true
);
);
Sign up or log in
StackExchange.ready(function ()
StackExchange.helpers.onClickDraftSave('#login-link');
);
Sign up using Google
Sign up using Facebook
Sign up using Email and Password
Post as a guest
Required, but never shown
StackExchange.ready(
function ()
StackExchange.openid.initPostLogin('.new-post-login', 'https%3a%2f%2fdatascience.stackexchange.com%2fquestions%2f48294%2fregression-vs-random-forest-combination-of-features%23new-answer', 'question_page');
);
Post as a guest
Required, but never shown
3 Answers
3
active
oldest
votes
3 Answers
3
active
oldest
votes
active
oldest
votes
active
oldest
votes
$begingroup$
I think it is true. Tree based algorithms especially the ones with multiple trees has the capability of capturing different feature interactions. Please see this article from xgboost official documentation and this discussion. You can say it's a perk of being a non parametric model (trees are non parametric and linear regression is not). I hope this will shed some light on this thought.
$endgroup$
$begingroup$
(+1) As an example,Tree 1 works with features (A, B) and gives 80% accuracy, Tree 2 works with features (C, D) and gives 60%. A boosting algorithm puts more weight on Tree 1, thus effectively favors f(A, B) over g(C, D).
$endgroup$
– Esmailian
Mar 31 at 19:14
$begingroup$
Thank you for your answer. However, to be honest I would like a more in depth answer. To start with, my second question is still unanswered I think: "Also if it true then is it about any kind of combination of features (e.g. X*W, X+W+Z etc) or only for some specific ones (e.g. X+W)?"
$endgroup$
– Poete Maudit
Apr 1 at 10:49
$begingroup$
Please refer this link ( mariofilho.com/can-gradient-boosting-learn-simple-arithmetic ) . This article talks about how boosting trees can model arithmetic operations like X*W, X/W, etc. Theoretically, it is possible. Trees are like neural networks, they are universal approximator (Theoretically). And I am stressing on the word Theoretically.
$endgroup$
– tam
Apr 1 at 11:05
$begingroup$
Ok thank you for this too. However, to start with both the other people here are claiming the opposite than you so it is quite difficult for me to draw a definite conclusion.
$endgroup$
– Poete Maudit
Apr 1 at 11:26
$begingroup$
Also by the way at your answer you are saying "... has the capability of capturing different feature interactions". However, my question is whether is built-in in random forest (or in boosting algos). In a sense, linear regression also has the "capability" of doing this but exactly you will have to programme it i.e. add some lines of code where you are adding, multiplying some of the features etc.
$endgroup$
– Poete Maudit
Apr 1 at 14:04
|
show 1 more comment
$begingroup$
I think it is true. Tree based algorithms especially the ones with multiple trees has the capability of capturing different feature interactions. Please see this article from xgboost official documentation and this discussion. You can say it's a perk of being a non parametric model (trees are non parametric and linear regression is not). I hope this will shed some light on this thought.
$endgroup$
$begingroup$
(+1) As an example,Tree 1 works with features (A, B) and gives 80% accuracy, Tree 2 works with features (C, D) and gives 60%. A boosting algorithm puts more weight on Tree 1, thus effectively favors f(A, B) over g(C, D).
$endgroup$
– Esmailian
Mar 31 at 19:14
$begingroup$
Thank you for your answer. However, to be honest I would like a more in depth answer. To start with, my second question is still unanswered I think: "Also if it true then is it about any kind of combination of features (e.g. X*W, X+W+Z etc) or only for some specific ones (e.g. X+W)?"
$endgroup$
– Poete Maudit
Apr 1 at 10:49
$begingroup$
Please refer this link ( mariofilho.com/can-gradient-boosting-learn-simple-arithmetic ) . This article talks about how boosting trees can model arithmetic operations like X*W, X/W, etc. Theoretically, it is possible. Trees are like neural networks, they are universal approximator (Theoretically). And I am stressing on the word Theoretically.
$endgroup$
– tam
Apr 1 at 11:05
$begingroup$
Ok thank you for this too. However, to start with both the other people here are claiming the opposite than you so it is quite difficult for me to draw a definite conclusion.
$endgroup$
– Poete Maudit
Apr 1 at 11:26
$begingroup$
Also by the way at your answer you are saying "... has the capability of capturing different feature interactions". However, my question is whether is built-in in random forest (or in boosting algos). In a sense, linear regression also has the "capability" of doing this but exactly you will have to programme it i.e. add some lines of code where you are adding, multiplying some of the features etc.
$endgroup$
– Poete Maudit
Apr 1 at 14:04
|
show 1 more comment
$begingroup$
I think it is true. Tree based algorithms especially the ones with multiple trees has the capability of capturing different feature interactions. Please see this article from xgboost official documentation and this discussion. You can say it's a perk of being a non parametric model (trees are non parametric and linear regression is not). I hope this will shed some light on this thought.
$endgroup$
I think it is true. Tree based algorithms especially the ones with multiple trees has the capability of capturing different feature interactions. Please see this article from xgboost official documentation and this discussion. You can say it's a perk of being a non parametric model (trees are non parametric and linear regression is not). I hope this will shed some light on this thought.
edited Mar 31 at 18:06
answered Mar 31 at 18:01
tamtam
1014
1014
$begingroup$
(+1) As an example,Tree 1 works with features (A, B) and gives 80% accuracy, Tree 2 works with features (C, D) and gives 60%. A boosting algorithm puts more weight on Tree 1, thus effectively favors f(A, B) over g(C, D).
$endgroup$
– Esmailian
Mar 31 at 19:14
$begingroup$
Thank you for your answer. However, to be honest I would like a more in depth answer. To start with, my second question is still unanswered I think: "Also if it true then is it about any kind of combination of features (e.g. X*W, X+W+Z etc) or only for some specific ones (e.g. X+W)?"
$endgroup$
– Poete Maudit
Apr 1 at 10:49
$begingroup$
Please refer this link ( mariofilho.com/can-gradient-boosting-learn-simple-arithmetic ) . This article talks about how boosting trees can model arithmetic operations like X*W, X/W, etc. Theoretically, it is possible. Trees are like neural networks, they are universal approximator (Theoretically). And I am stressing on the word Theoretically.
$endgroup$
– tam
Apr 1 at 11:05
$begingroup$
Ok thank you for this too. However, to start with both the other people here are claiming the opposite than you so it is quite difficult for me to draw a definite conclusion.
$endgroup$
– Poete Maudit
Apr 1 at 11:26
$begingroup$
Also by the way at your answer you are saying "... has the capability of capturing different feature interactions". However, my question is whether is built-in in random forest (or in boosting algos). In a sense, linear regression also has the "capability" of doing this but exactly you will have to programme it i.e. add some lines of code where you are adding, multiplying some of the features etc.
$endgroup$
– Poete Maudit
Apr 1 at 14:04
|
show 1 more comment
$begingroup$
(+1) As an example,Tree 1 works with features (A, B) and gives 80% accuracy, Tree 2 works with features (C, D) and gives 60%. A boosting algorithm puts more weight on Tree 1, thus effectively favors f(A, B) over g(C, D).
$endgroup$
– Esmailian
Mar 31 at 19:14
$begingroup$
Thank you for your answer. However, to be honest I would like a more in depth answer. To start with, my second question is still unanswered I think: "Also if it true then is it about any kind of combination of features (e.g. X*W, X+W+Z etc) or only for some specific ones (e.g. X+W)?"
$endgroup$
– Poete Maudit
Apr 1 at 10:49
$begingroup$
Please refer this link ( mariofilho.com/can-gradient-boosting-learn-simple-arithmetic ) . This article talks about how boosting trees can model arithmetic operations like X*W, X/W, etc. Theoretically, it is possible. Trees are like neural networks, they are universal approximator (Theoretically). And I am stressing on the word Theoretically.
$endgroup$
– tam
Apr 1 at 11:05
$begingroup$
Ok thank you for this too. However, to start with both the other people here are claiming the opposite than you so it is quite difficult for me to draw a definite conclusion.
$endgroup$
– Poete Maudit
Apr 1 at 11:26
$begingroup$
Also by the way at your answer you are saying "... has the capability of capturing different feature interactions". However, my question is whether is built-in in random forest (or in boosting algos). In a sense, linear regression also has the "capability" of doing this but exactly you will have to programme it i.e. add some lines of code where you are adding, multiplying some of the features etc.
$endgroup$
– Poete Maudit
Apr 1 at 14:04
$begingroup$
(+1) As an example,Tree 1 works with features (A, B) and gives 80% accuracy, Tree 2 works with features (C, D) and gives 60%. A boosting algorithm puts more weight on Tree 1, thus effectively favors f(A, B) over g(C, D).
$endgroup$
– Esmailian
Mar 31 at 19:14
$begingroup$
(+1) As an example,Tree 1 works with features (A, B) and gives 80% accuracy, Tree 2 works with features (C, D) and gives 60%. A boosting algorithm puts more weight on Tree 1, thus effectively favors f(A, B) over g(C, D).
$endgroup$
– Esmailian
Mar 31 at 19:14
$begingroup$
Thank you for your answer. However, to be honest I would like a more in depth answer. To start with, my second question is still unanswered I think: "Also if it true then is it about any kind of combination of features (e.g. X*W, X+W+Z etc) or only for some specific ones (e.g. X+W)?"
$endgroup$
– Poete Maudit
Apr 1 at 10:49
$begingroup$
Thank you for your answer. However, to be honest I would like a more in depth answer. To start with, my second question is still unanswered I think: "Also if it true then is it about any kind of combination of features (e.g. X*W, X+W+Z etc) or only for some specific ones (e.g. X+W)?"
$endgroup$
– Poete Maudit
Apr 1 at 10:49
$begingroup$
Please refer this link ( mariofilho.com/can-gradient-boosting-learn-simple-arithmetic ) . This article talks about how boosting trees can model arithmetic operations like X*W, X/W, etc. Theoretically, it is possible. Trees are like neural networks, they are universal approximator (Theoretically). And I am stressing on the word Theoretically.
$endgroup$
– tam
Apr 1 at 11:05
$begingroup$
Please refer this link ( mariofilho.com/can-gradient-boosting-learn-simple-arithmetic ) . This article talks about how boosting trees can model arithmetic operations like X*W, X/W, etc. Theoretically, it is possible. Trees are like neural networks, they are universal approximator (Theoretically). And I am stressing on the word Theoretically.
$endgroup$
– tam
Apr 1 at 11:05
$begingroup$
Ok thank you for this too. However, to start with both the other people here are claiming the opposite than you so it is quite difficult for me to draw a definite conclusion.
$endgroup$
– Poete Maudit
Apr 1 at 11:26
$begingroup$
Ok thank you for this too. However, to start with both the other people here are claiming the opposite than you so it is quite difficult for me to draw a definite conclusion.
$endgroup$
– Poete Maudit
Apr 1 at 11:26
$begingroup$
Also by the way at your answer you are saying "... has the capability of capturing different feature interactions". However, my question is whether is built-in in random forest (or in boosting algos). In a sense, linear regression also has the "capability" of doing this but exactly you will have to programme it i.e. add some lines of code where you are adding, multiplying some of the features etc.
$endgroup$
– Poete Maudit
Apr 1 at 14:04
$begingroup$
Also by the way at your answer you are saying "... has the capability of capturing different feature interactions". However, my question is whether is built-in in random forest (or in boosting algos). In a sense, linear regression also has the "capability" of doing this but exactly you will have to programme it i.e. add some lines of code where you are adding, multiplying some of the features etc.
$endgroup$
– Poete Maudit
Apr 1 at 14:04
|
show 1 more comment
$begingroup$
I would say it is not true as Random forests which are made up of decision trees does perform feature selection but they do not perform feature engineering (feature selection is different from feature engineering). Decision trees use a metric called Information gain (which is total entropy minus the weighted entropy) as per which useful features are separated from bad features. Simply to say whichever feature exhibit the highest information gain on this iteration is chosen as the node on which the tree on this iteration is split or you can say which feature reduces the entropy(aka randomness) the most in this iteration is chosen as the node upon which the tree is split on this iteration. So if you data is text, trees are split upon words. If your data is real valued numbers, tree is split upon that. Hope it helps
For more details check this
$endgroup$
$begingroup$
Thank you for your answer. However, to be honest I would like a more in depth answer. To start with, my second question is still unanswered I think: "Also if it true then is it about any kind of combination of features (e.g. X*W, X+W+Z etc) or only for some specific ones (e.g. X+W)?"
$endgroup$
– Poete Maudit
Apr 1 at 10:49
$begingroup$
Yes as said in my previous answer, decision trees cannot perform feature engineering by themselves. They pick the right feature based on information gain which is called as the feature selection. So (X+W), (X*W) or any sort of simple or complex feature engineered features are not possible in case of tree based models. So answer to your second question is "No, Tree based methods cannot and will not perform feature engineering on their own". Hope it's clear
$endgroup$
– karthikeyan mg
Apr 1 at 11:15
$begingroup$
Now it is significantly clearer because your starting phrase "I would say it is partly true as Random forests..." confuses things a bit. So basically at my question your answer is "no it is not true; random forest does not take into account the combination of features e.g. X+W etc". It would be good to modify a bit your post because this is not evident.
$endgroup$
– Poete Maudit
Apr 1 at 11:23
$begingroup$
However, I will have to see some evidence on why the boosting algorithms do this while the bagging algorithms do not. Also, in the case of the boosting algorithms how the algorithm chooses which of the various combinations to test?
$endgroup$
– Poete Maudit
Apr 1 at 11:25
$begingroup$
Thanks for the suggestion, I've made the changes. And regarding your last comment, just to be clear, random forests comes under bagging algos and gbdt, xgboost comes under boosting. I'd suggest you draft another question explaining your last comment in detail along with your thoughts and understanding and link the question here, We will try our best to help you! Cheers
$endgroup$
– karthikeyan mg
Apr 1 at 11:48
|
show 1 more comment
$begingroup$
I would say it is not true as Random forests which are made up of decision trees does perform feature selection but they do not perform feature engineering (feature selection is different from feature engineering). Decision trees use a metric called Information gain (which is total entropy minus the weighted entropy) as per which useful features are separated from bad features. Simply to say whichever feature exhibit the highest information gain on this iteration is chosen as the node on which the tree on this iteration is split or you can say which feature reduces the entropy(aka randomness) the most in this iteration is chosen as the node upon which the tree is split on this iteration. So if you data is text, trees are split upon words. If your data is real valued numbers, tree is split upon that. Hope it helps
For more details check this
$endgroup$
$begingroup$
Thank you for your answer. However, to be honest I would like a more in depth answer. To start with, my second question is still unanswered I think: "Also if it true then is it about any kind of combination of features (e.g. X*W, X+W+Z etc) or only for some specific ones (e.g. X+W)?"
$endgroup$
– Poete Maudit
Apr 1 at 10:49
$begingroup$
Yes as said in my previous answer, decision trees cannot perform feature engineering by themselves. They pick the right feature based on information gain which is called as the feature selection. So (X+W), (X*W) or any sort of simple or complex feature engineered features are not possible in case of tree based models. So answer to your second question is "No, Tree based methods cannot and will not perform feature engineering on their own". Hope it's clear
$endgroup$
– karthikeyan mg
Apr 1 at 11:15
$begingroup$
Now it is significantly clearer because your starting phrase "I would say it is partly true as Random forests..." confuses things a bit. So basically at my question your answer is "no it is not true; random forest does not take into account the combination of features e.g. X+W etc". It would be good to modify a bit your post because this is not evident.
$endgroup$
– Poete Maudit
Apr 1 at 11:23
$begingroup$
However, I will have to see some evidence on why the boosting algorithms do this while the bagging algorithms do not. Also, in the case of the boosting algorithms how the algorithm chooses which of the various combinations to test?
$endgroup$
– Poete Maudit
Apr 1 at 11:25
$begingroup$
Thanks for the suggestion, I've made the changes. And regarding your last comment, just to be clear, random forests comes under bagging algos and gbdt, xgboost comes under boosting. I'd suggest you draft another question explaining your last comment in detail along with your thoughts and understanding and link the question here, We will try our best to help you! Cheers
$endgroup$
– karthikeyan mg
Apr 1 at 11:48
|
show 1 more comment
$begingroup$
I would say it is not true as Random forests which are made up of decision trees does perform feature selection but they do not perform feature engineering (feature selection is different from feature engineering). Decision trees use a metric called Information gain (which is total entropy minus the weighted entropy) as per which useful features are separated from bad features. Simply to say whichever feature exhibit the highest information gain on this iteration is chosen as the node on which the tree on this iteration is split or you can say which feature reduces the entropy(aka randomness) the most in this iteration is chosen as the node upon which the tree is split on this iteration. So if you data is text, trees are split upon words. If your data is real valued numbers, tree is split upon that. Hope it helps
For more details check this
$endgroup$
I would say it is not true as Random forests which are made up of decision trees does perform feature selection but they do not perform feature engineering (feature selection is different from feature engineering). Decision trees use a metric called Information gain (which is total entropy minus the weighted entropy) as per which useful features are separated from bad features. Simply to say whichever feature exhibit the highest information gain on this iteration is chosen as the node on which the tree on this iteration is split or you can say which feature reduces the entropy(aka randomness) the most in this iteration is chosen as the node upon which the tree is split on this iteration. So if you data is text, trees are split upon words. If your data is real valued numbers, tree is split upon that. Hope it helps
For more details check this
edited Apr 1 at 11:31
answered Mar 31 at 15:37


karthikeyan mgkarthikeyan mg
305111
305111
$begingroup$
Thank you for your answer. However, to be honest I would like a more in depth answer. To start with, my second question is still unanswered I think: "Also if it true then is it about any kind of combination of features (e.g. X*W, X+W+Z etc) or only for some specific ones (e.g. X+W)?"
$endgroup$
– Poete Maudit
Apr 1 at 10:49
$begingroup$
Yes as said in my previous answer, decision trees cannot perform feature engineering by themselves. They pick the right feature based on information gain which is called as the feature selection. So (X+W), (X*W) or any sort of simple or complex feature engineered features are not possible in case of tree based models. So answer to your second question is "No, Tree based methods cannot and will not perform feature engineering on their own". Hope it's clear
$endgroup$
– karthikeyan mg
Apr 1 at 11:15
$begingroup$
Now it is significantly clearer because your starting phrase "I would say it is partly true as Random forests..." confuses things a bit. So basically at my question your answer is "no it is not true; random forest does not take into account the combination of features e.g. X+W etc". It would be good to modify a bit your post because this is not evident.
$endgroup$
– Poete Maudit
Apr 1 at 11:23
$begingroup$
However, I will have to see some evidence on why the boosting algorithms do this while the bagging algorithms do not. Also, in the case of the boosting algorithms how the algorithm chooses which of the various combinations to test?
$endgroup$
– Poete Maudit
Apr 1 at 11:25
$begingroup$
Thanks for the suggestion, I've made the changes. And regarding your last comment, just to be clear, random forests comes under bagging algos and gbdt, xgboost comes under boosting. I'd suggest you draft another question explaining your last comment in detail along with your thoughts and understanding and link the question here, We will try our best to help you! Cheers
$endgroup$
– karthikeyan mg
Apr 1 at 11:48
|
show 1 more comment
$begingroup$
Thank you for your answer. However, to be honest I would like a more in depth answer. To start with, my second question is still unanswered I think: "Also if it true then is it about any kind of combination of features (e.g. X*W, X+W+Z etc) or only for some specific ones (e.g. X+W)?"
$endgroup$
– Poete Maudit
Apr 1 at 10:49
$begingroup$
Yes as said in my previous answer, decision trees cannot perform feature engineering by themselves. They pick the right feature based on information gain which is called as the feature selection. So (X+W), (X*W) or any sort of simple or complex feature engineered features are not possible in case of tree based models. So answer to your second question is "No, Tree based methods cannot and will not perform feature engineering on their own". Hope it's clear
$endgroup$
– karthikeyan mg
Apr 1 at 11:15
$begingroup$
Now it is significantly clearer because your starting phrase "I would say it is partly true as Random forests..." confuses things a bit. So basically at my question your answer is "no it is not true; random forest does not take into account the combination of features e.g. X+W etc". It would be good to modify a bit your post because this is not evident.
$endgroup$
– Poete Maudit
Apr 1 at 11:23
$begingroup$
However, I will have to see some evidence on why the boosting algorithms do this while the bagging algorithms do not. Also, in the case of the boosting algorithms how the algorithm chooses which of the various combinations to test?
$endgroup$
– Poete Maudit
Apr 1 at 11:25
$begingroup$
Thanks for the suggestion, I've made the changes. And regarding your last comment, just to be clear, random forests comes under bagging algos and gbdt, xgboost comes under boosting. I'd suggest you draft another question explaining your last comment in detail along with your thoughts and understanding and link the question here, We will try our best to help you! Cheers
$endgroup$
– karthikeyan mg
Apr 1 at 11:48
$begingroup$
Thank you for your answer. However, to be honest I would like a more in depth answer. To start with, my second question is still unanswered I think: "Also if it true then is it about any kind of combination of features (e.g. X*W, X+W+Z etc) or only for some specific ones (e.g. X+W)?"
$endgroup$
– Poete Maudit
Apr 1 at 10:49
$begingroup$
Thank you for your answer. However, to be honest I would like a more in depth answer. To start with, my second question is still unanswered I think: "Also if it true then is it about any kind of combination of features (e.g. X*W, X+W+Z etc) or only for some specific ones (e.g. X+W)?"
$endgroup$
– Poete Maudit
Apr 1 at 10:49
$begingroup$
Yes as said in my previous answer, decision trees cannot perform feature engineering by themselves. They pick the right feature based on information gain which is called as the feature selection. So (X+W), (X*W) or any sort of simple or complex feature engineered features are not possible in case of tree based models. So answer to your second question is "No, Tree based methods cannot and will not perform feature engineering on their own". Hope it's clear
$endgroup$
– karthikeyan mg
Apr 1 at 11:15
$begingroup$
Yes as said in my previous answer, decision trees cannot perform feature engineering by themselves. They pick the right feature based on information gain which is called as the feature selection. So (X+W), (X*W) or any sort of simple or complex feature engineered features are not possible in case of tree based models. So answer to your second question is "No, Tree based methods cannot and will not perform feature engineering on their own". Hope it's clear
$endgroup$
– karthikeyan mg
Apr 1 at 11:15
$begingroup$
Now it is significantly clearer because your starting phrase "I would say it is partly true as Random forests..." confuses things a bit. So basically at my question your answer is "no it is not true; random forest does not take into account the combination of features e.g. X+W etc". It would be good to modify a bit your post because this is not evident.
$endgroup$
– Poete Maudit
Apr 1 at 11:23
$begingroup$
Now it is significantly clearer because your starting phrase "I would say it is partly true as Random forests..." confuses things a bit. So basically at my question your answer is "no it is not true; random forest does not take into account the combination of features e.g. X+W etc". It would be good to modify a bit your post because this is not evident.
$endgroup$
– Poete Maudit
Apr 1 at 11:23
$begingroup$
However, I will have to see some evidence on why the boosting algorithms do this while the bagging algorithms do not. Also, in the case of the boosting algorithms how the algorithm chooses which of the various combinations to test?
$endgroup$
– Poete Maudit
Apr 1 at 11:25
$begingroup$
However, I will have to see some evidence on why the boosting algorithms do this while the bagging algorithms do not. Also, in the case of the boosting algorithms how the algorithm chooses which of the various combinations to test?
$endgroup$
– Poete Maudit
Apr 1 at 11:25
$begingroup$
Thanks for the suggestion, I've made the changes. And regarding your last comment, just to be clear, random forests comes under bagging algos and gbdt, xgboost comes under boosting. I'd suggest you draft another question explaining your last comment in detail along with your thoughts and understanding and link the question here, We will try our best to help you! Cheers
$endgroup$
– karthikeyan mg
Apr 1 at 11:48
$begingroup$
Thanks for the suggestion, I've made the changes. And regarding your last comment, just to be clear, random forests comes under bagging algos and gbdt, xgboost comes under boosting. I'd suggest you draft another question explaining your last comment in detail along with your thoughts and understanding and link the question here, We will try our best to help you! Cheers
$endgroup$
– karthikeyan mg
Apr 1 at 11:48
|
show 1 more comment
$begingroup$
The statement "it tests combination of features" is not true. It tests individual features. However, a tree can approximate any continuous function $f$ over training points, since it is a universal approximator just like neural networks.
In Random Forest (or Decision Tree, or Regression Tree), individual features are compared to each other, not a combination of them, then the most informative individual is peaked to split a leaf. Therefore, there is no notion of "better combination" in the whole process.
Furthermore, Random Forest is a bagging algorithm which does not favor the randomly-built trees over each other, they all have the same weight in the aggregated output.
It is worth noting that "Rotation forest" first applies PCA to features, which means each new feature is a linear combination of original features. However, this does not count since the same pre-processing can be used for any other method too.
EDIT:
@tam provided a counter-example for XGBoost, which is not the same as Random Forest. However, the issue is the same for XGBoost. Its learning process comes down to splitting each leaf greadily based on a single feature instead of selecting the best combination of features among a set of combinations, or the best tree among a set of trees.
From this explanation, you can see that The Structure Score is defined for a tree (which is a function) based on the first- and second-order derivatives of loss function in each leaf $j$ ($G_j$ and $H_j$ respectively) summed over all $T$ leaves, i.e.
$$textobj^*=-frac12 sum_j=1^TfracG_jH_j + lambda + gamma T$$
However, the optimization process greedily splits a leaf using the best individual feature that gives the highest gain in $textobj^*$.
A tree $t$ is built by greedily minimizing the loss, i.e. branching on the best individual feature, and when the tree is built, process goes to create the next tree $t+1$ in the same way, and so on.
Here is the key quote from XGBoost paper:
This score is like the impurity score for evaluating decision trees,
except that it is derived for a wider range of objective functions [..] Normally it is impossible to enumerate all the possible tree
structures q. A greedy algorithm that starts from a single leaf and
iteratively adds branches to the tree is used instead.
In summary:
Although a tree represents a combination of features (a function), but
none of XGBoost and Random Forest are selecting between functions.
They build and aggregate multiple functions by greedily favoring individual
features.
$endgroup$
$begingroup$
Thank you for your answer. My post triggered some opposing views and now in this sense I do not know yet which side to take. By the way, my impression is that the remark of @tam is not really directly to the point. The fact that tree boosting algorithms favor f(X, Y) over g(Y, W) does not necessarily mean that they take into account the combination of the features in the sense of e.g. X+W but they simply favor groups of features over other groups of features. Thus, not combination of features but groups of features (if I am not missing anything).
$endgroup$
– Poete Maudit
Apr 1 at 10:56
$begingroup$
@PoeteMaudit I added an example.
$endgroup$
– Esmailian
Apr 1 at 11:04
$begingroup$
Cool, thank you. However, I will have to see some evidence on why the boosting algorithms do this while the bagging algorithms do not. Also, in the case of the boosting algorithms how the algorithm chooses which of the various combinations to test?
$endgroup$
– Poete Maudit
Apr 1 at 11:25
1
$begingroup$
So your answer to my question is that "Note that, a tree can approximate any continuous function f over training points, since it is a universal approximator just like neural networks."? If so then this is interesting.
$endgroup$
– Poete Maudit
Apr 1 at 13:55
add a comment |
$begingroup$
The statement "it tests combination of features" is not true. It tests individual features. However, a tree can approximate any continuous function $f$ over training points, since it is a universal approximator just like neural networks.
In Random Forest (or Decision Tree, or Regression Tree), individual features are compared to each other, not a combination of them, then the most informative individual is peaked to split a leaf. Therefore, there is no notion of "better combination" in the whole process.
Furthermore, Random Forest is a bagging algorithm which does not favor the randomly-built trees over each other, they all have the same weight in the aggregated output.
It is worth noting that "Rotation forest" first applies PCA to features, which means each new feature is a linear combination of original features. However, this does not count since the same pre-processing can be used for any other method too.
EDIT:
@tam provided a counter-example for XGBoost, which is not the same as Random Forest. However, the issue is the same for XGBoost. Its learning process comes down to splitting each leaf greadily based on a single feature instead of selecting the best combination of features among a set of combinations, or the best tree among a set of trees.
From this explanation, you can see that The Structure Score is defined for a tree (which is a function) based on the first- and second-order derivatives of loss function in each leaf $j$ ($G_j$ and $H_j$ respectively) summed over all $T$ leaves, i.e.
$$textobj^*=-frac12 sum_j=1^TfracG_jH_j + lambda + gamma T$$
However, the optimization process greedily splits a leaf using the best individual feature that gives the highest gain in $textobj^*$.
A tree $t$ is built by greedily minimizing the loss, i.e. branching on the best individual feature, and when the tree is built, process goes to create the next tree $t+1$ in the same way, and so on.
Here is the key quote from XGBoost paper:
This score is like the impurity score for evaluating decision trees,
except that it is derived for a wider range of objective functions [..] Normally it is impossible to enumerate all the possible tree
structures q. A greedy algorithm that starts from a single leaf and
iteratively adds branches to the tree is used instead.
In summary:
Although a tree represents a combination of features (a function), but
none of XGBoost and Random Forest are selecting between functions.
They build and aggregate multiple functions by greedily favoring individual
features.
$endgroup$
$begingroup$
Thank you for your answer. My post triggered some opposing views and now in this sense I do not know yet which side to take. By the way, my impression is that the remark of @tam is not really directly to the point. The fact that tree boosting algorithms favor f(X, Y) over g(Y, W) does not necessarily mean that they take into account the combination of the features in the sense of e.g. X+W but they simply favor groups of features over other groups of features. Thus, not combination of features but groups of features (if I am not missing anything).
$endgroup$
– Poete Maudit
Apr 1 at 10:56
$begingroup$
@PoeteMaudit I added an example.
$endgroup$
– Esmailian
Apr 1 at 11:04
$begingroup$
Cool, thank you. However, I will have to see some evidence on why the boosting algorithms do this while the bagging algorithms do not. Also, in the case of the boosting algorithms how the algorithm chooses which of the various combinations to test?
$endgroup$
– Poete Maudit
Apr 1 at 11:25
1
$begingroup$
So your answer to my question is that "Note that, a tree can approximate any continuous function f over training points, since it is a universal approximator just like neural networks."? If so then this is interesting.
$endgroup$
– Poete Maudit
Apr 1 at 13:55
add a comment |
$begingroup$
The statement "it tests combination of features" is not true. It tests individual features. However, a tree can approximate any continuous function $f$ over training points, since it is a universal approximator just like neural networks.
In Random Forest (or Decision Tree, or Regression Tree), individual features are compared to each other, not a combination of them, then the most informative individual is peaked to split a leaf. Therefore, there is no notion of "better combination" in the whole process.
Furthermore, Random Forest is a bagging algorithm which does not favor the randomly-built trees over each other, they all have the same weight in the aggregated output.
It is worth noting that "Rotation forest" first applies PCA to features, which means each new feature is a linear combination of original features. However, this does not count since the same pre-processing can be used for any other method too.
EDIT:
@tam provided a counter-example for XGBoost, which is not the same as Random Forest. However, the issue is the same for XGBoost. Its learning process comes down to splitting each leaf greadily based on a single feature instead of selecting the best combination of features among a set of combinations, or the best tree among a set of trees.
From this explanation, you can see that The Structure Score is defined for a tree (which is a function) based on the first- and second-order derivatives of loss function in each leaf $j$ ($G_j$ and $H_j$ respectively) summed over all $T$ leaves, i.e.
$$textobj^*=-frac12 sum_j=1^TfracG_jH_j + lambda + gamma T$$
However, the optimization process greedily splits a leaf using the best individual feature that gives the highest gain in $textobj^*$.
A tree $t$ is built by greedily minimizing the loss, i.e. branching on the best individual feature, and when the tree is built, process goes to create the next tree $t+1$ in the same way, and so on.
Here is the key quote from XGBoost paper:
This score is like the impurity score for evaluating decision trees,
except that it is derived for a wider range of objective functions [..] Normally it is impossible to enumerate all the possible tree
structures q. A greedy algorithm that starts from a single leaf and
iteratively adds branches to the tree is used instead.
In summary:
Although a tree represents a combination of features (a function), but
none of XGBoost and Random Forest are selecting between functions.
They build and aggregate multiple functions by greedily favoring individual
features.
$endgroup$
The statement "it tests combination of features" is not true. It tests individual features. However, a tree can approximate any continuous function $f$ over training points, since it is a universal approximator just like neural networks.
In Random Forest (or Decision Tree, or Regression Tree), individual features are compared to each other, not a combination of them, then the most informative individual is peaked to split a leaf. Therefore, there is no notion of "better combination" in the whole process.
Furthermore, Random Forest is a bagging algorithm which does not favor the randomly-built trees over each other, they all have the same weight in the aggregated output.
It is worth noting that "Rotation forest" first applies PCA to features, which means each new feature is a linear combination of original features. However, this does not count since the same pre-processing can be used for any other method too.
EDIT:
@tam provided a counter-example for XGBoost, which is not the same as Random Forest. However, the issue is the same for XGBoost. Its learning process comes down to splitting each leaf greadily based on a single feature instead of selecting the best combination of features among a set of combinations, or the best tree among a set of trees.
From this explanation, you can see that The Structure Score is defined for a tree (which is a function) based on the first- and second-order derivatives of loss function in each leaf $j$ ($G_j$ and $H_j$ respectively) summed over all $T$ leaves, i.e.
$$textobj^*=-frac12 sum_j=1^TfracG_jH_j + lambda + gamma T$$
However, the optimization process greedily splits a leaf using the best individual feature that gives the highest gain in $textobj^*$.
A tree $t$ is built by greedily minimizing the loss, i.e. branching on the best individual feature, and when the tree is built, process goes to create the next tree $t+1$ in the same way, and so on.
Here is the key quote from XGBoost paper:
This score is like the impurity score for evaluating decision trees,
except that it is derived for a wider range of objective functions [..] Normally it is impossible to enumerate all the possible tree
structures q. A greedy algorithm that starts from a single leaf and
iteratively adds branches to the tree is used instead.
In summary:
Although a tree represents a combination of features (a function), but
none of XGBoost and Random Forest are selecting between functions.
They build and aggregate multiple functions by greedily favoring individual
features.
edited Apr 5 at 10:09
answered Mar 31 at 16:20
EsmailianEsmailian
3,206320
3,206320
$begingroup$
Thank you for your answer. My post triggered some opposing views and now in this sense I do not know yet which side to take. By the way, my impression is that the remark of @tam is not really directly to the point. The fact that tree boosting algorithms favor f(X, Y) over g(Y, W) does not necessarily mean that they take into account the combination of the features in the sense of e.g. X+W but they simply favor groups of features over other groups of features. Thus, not combination of features but groups of features (if I am not missing anything).
$endgroup$
– Poete Maudit
Apr 1 at 10:56
$begingroup$
@PoeteMaudit I added an example.
$endgroup$
– Esmailian
Apr 1 at 11:04
$begingroup$
Cool, thank you. However, I will have to see some evidence on why the boosting algorithms do this while the bagging algorithms do not. Also, in the case of the boosting algorithms how the algorithm chooses which of the various combinations to test?
$endgroup$
– Poete Maudit
Apr 1 at 11:25
1
$begingroup$
So your answer to my question is that "Note that, a tree can approximate any continuous function f over training points, since it is a universal approximator just like neural networks."? If so then this is interesting.
$endgroup$
– Poete Maudit
Apr 1 at 13:55
add a comment |
$begingroup$
Thank you for your answer. My post triggered some opposing views and now in this sense I do not know yet which side to take. By the way, my impression is that the remark of @tam is not really directly to the point. The fact that tree boosting algorithms favor f(X, Y) over g(Y, W) does not necessarily mean that they take into account the combination of the features in the sense of e.g. X+W but they simply favor groups of features over other groups of features. Thus, not combination of features but groups of features (if I am not missing anything).
$endgroup$
– Poete Maudit
Apr 1 at 10:56
$begingroup$
@PoeteMaudit I added an example.
$endgroup$
– Esmailian
Apr 1 at 11:04
$begingroup$
Cool, thank you. However, I will have to see some evidence on why the boosting algorithms do this while the bagging algorithms do not. Also, in the case of the boosting algorithms how the algorithm chooses which of the various combinations to test?
$endgroup$
– Poete Maudit
Apr 1 at 11:25
1
$begingroup$
So your answer to my question is that "Note that, a tree can approximate any continuous function f over training points, since it is a universal approximator just like neural networks."? If so then this is interesting.
$endgroup$
– Poete Maudit
Apr 1 at 13:55
$begingroup$
Thank you for your answer. My post triggered some opposing views and now in this sense I do not know yet which side to take. By the way, my impression is that the remark of @tam is not really directly to the point. The fact that tree boosting algorithms favor f(X, Y) over g(Y, W) does not necessarily mean that they take into account the combination of the features in the sense of e.g. X+W but they simply favor groups of features over other groups of features. Thus, not combination of features but groups of features (if I am not missing anything).
$endgroup$
– Poete Maudit
Apr 1 at 10:56
$begingroup$
Thank you for your answer. My post triggered some opposing views and now in this sense I do not know yet which side to take. By the way, my impression is that the remark of @tam is not really directly to the point. The fact that tree boosting algorithms favor f(X, Y) over g(Y, W) does not necessarily mean that they take into account the combination of the features in the sense of e.g. X+W but they simply favor groups of features over other groups of features. Thus, not combination of features but groups of features (if I am not missing anything).
$endgroup$
– Poete Maudit
Apr 1 at 10:56
$begingroup$
@PoeteMaudit I added an example.
$endgroup$
– Esmailian
Apr 1 at 11:04
$begingroup$
@PoeteMaudit I added an example.
$endgroup$
– Esmailian
Apr 1 at 11:04
$begingroup$
Cool, thank you. However, I will have to see some evidence on why the boosting algorithms do this while the bagging algorithms do not. Also, in the case of the boosting algorithms how the algorithm chooses which of the various combinations to test?
$endgroup$
– Poete Maudit
Apr 1 at 11:25
$begingroup$
Cool, thank you. However, I will have to see some evidence on why the boosting algorithms do this while the bagging algorithms do not. Also, in the case of the boosting algorithms how the algorithm chooses which of the various combinations to test?
$endgroup$
– Poete Maudit
Apr 1 at 11:25
1
1
$begingroup$
So your answer to my question is that "Note that, a tree can approximate any continuous function f over training points, since it is a universal approximator just like neural networks."? If so then this is interesting.
$endgroup$
– Poete Maudit
Apr 1 at 13:55
$begingroup$
So your answer to my question is that "Note that, a tree can approximate any continuous function f over training points, since it is a universal approximator just like neural networks."? If so then this is interesting.
$endgroup$
– Poete Maudit
Apr 1 at 13:55
add a comment |
Thanks for contributing an answer to Data Science Stack Exchange!
- Please be sure to answer the question. Provide details and share your research!
But avoid …
- Asking for help, clarification, or responding to other answers.
- Making statements based on opinion; back them up with references or personal experience.
Use MathJax to format equations. MathJax reference.
To learn more, see our tips on writing great answers.
Sign up or log in
StackExchange.ready(function ()
StackExchange.helpers.onClickDraftSave('#login-link');
);
Sign up using Google
Sign up using Facebook
Sign up using Email and Password
Post as a guest
Required, but never shown
StackExchange.ready(
function ()
StackExchange.openid.initPostLogin('.new-post-login', 'https%3a%2f%2fdatascience.stackexchange.com%2fquestions%2f48294%2fregression-vs-random-forest-combination-of-features%23new-answer', 'question_page');
);
Post as a guest
Required, but never shown
Sign up or log in
StackExchange.ready(function ()
StackExchange.helpers.onClickDraftSave('#login-link');
);
Sign up using Google
Sign up using Facebook
Sign up using Email and Password
Post as a guest
Required, but never shown
Sign up or log in
StackExchange.ready(function ()
StackExchange.helpers.onClickDraftSave('#login-link');
);
Sign up using Google
Sign up using Facebook
Sign up using Email and Password
Post as a guest
Required, but never shown
Sign up or log in
StackExchange.ready(function ()
StackExchange.helpers.onClickDraftSave('#login-link');
);
Sign up using Google
Sign up using Facebook
Sign up using Email and Password
Sign up using Google
Sign up using Facebook
Sign up using Email and Password
Post as a guest
Required, but never shown
Required, but never shown
Required, but never shown
Required, but never shown
Required, but never shown
Required, but never shown
Required, but never shown
Required, but never shown
Required, but never shown
TwCV88ulf,OXJrgfIw60lAK1HmRgaQThqUj,JWT8rVWzMT UgUFxVIGrp,Fw,OXZXmcTPEq,0jL9LU6Ojo3m11g38g3VhVcZJQC4dCE